Lisa’s journey: Embracing Simcenter Amesim Recommender as a new simulation engineer
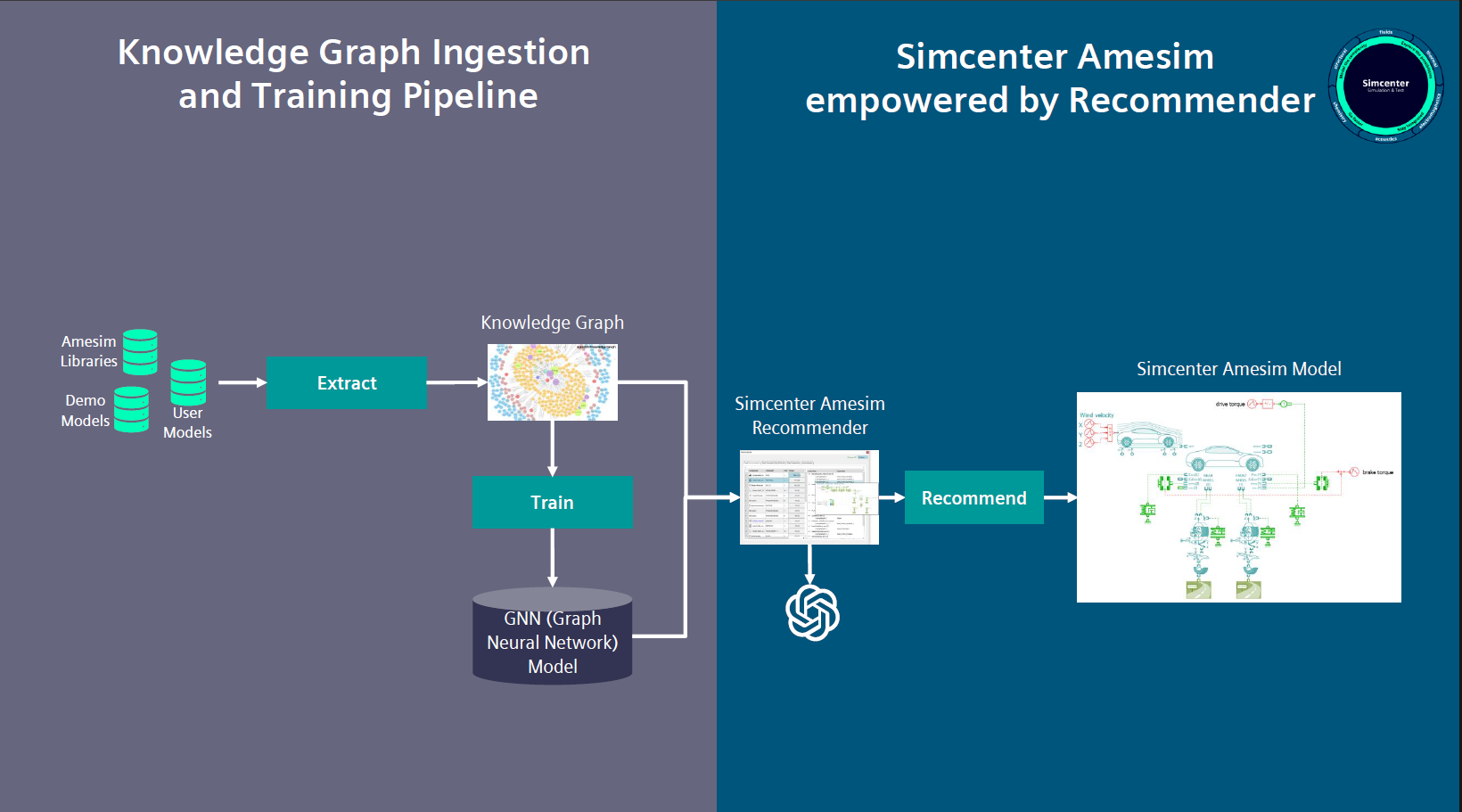
Introduction
Lisa1 is a recent computer science graduate. She is eager to begin her professional journey as a simulation engineer at X-Car2, a leading automobile provider. Her initial project involves using Simcenter Amesim to conduct a behavioral analysis of a new car concept design created by the architecture team. Simcenter Amesim is a simulation software that she has not yet had experience with. After completing the standard Simcenter Amesim training courses, she gained a fundamental understanding of how to utilize the tool. This included creating sketches, configuring submodels3 and parameters, as well as running simulations. Despite this, creating a simulation that meets the specific requirements of the architecture team remains a major challenge for her. She has learned about a tool called Simcenter Amesim Recommender4 that can provide guidance to both beginners and experts in the modeling process. She plans to give it a try. In this blog post, we will track Lisa’s progress and explore the benefits that engineers can enjoy by using this tool.
Training
X-Car has been a regular user of Simcenter Amesim for a decade. Several projects have utilized Simcenter Amesim to validate the designs of multiple generations of cars. There are thousands of models at different abstraction levels for previous car generations, which have been meticulously verified in accordance with the modeling team’s standards and design patterns. Although the Recommender shipped by Siemens has learned from standard demo models and best practices created by Siemens engineers, incorporating X-Car’s historical models and best practices requires training the Recommender. The training has already been done by Lisa’s IT administrator, who initiated the knowledge ingestion pipeline offered by the Recommender to transform the historical model database into a knowledge graph. Subsequently, a post-training was conducted on top of the knowledge graph database to train a model capable of predicting and ranking recommendations for the current design context. With this customized Recommender, Lisa gets an excellent starting point for her modeling journey.
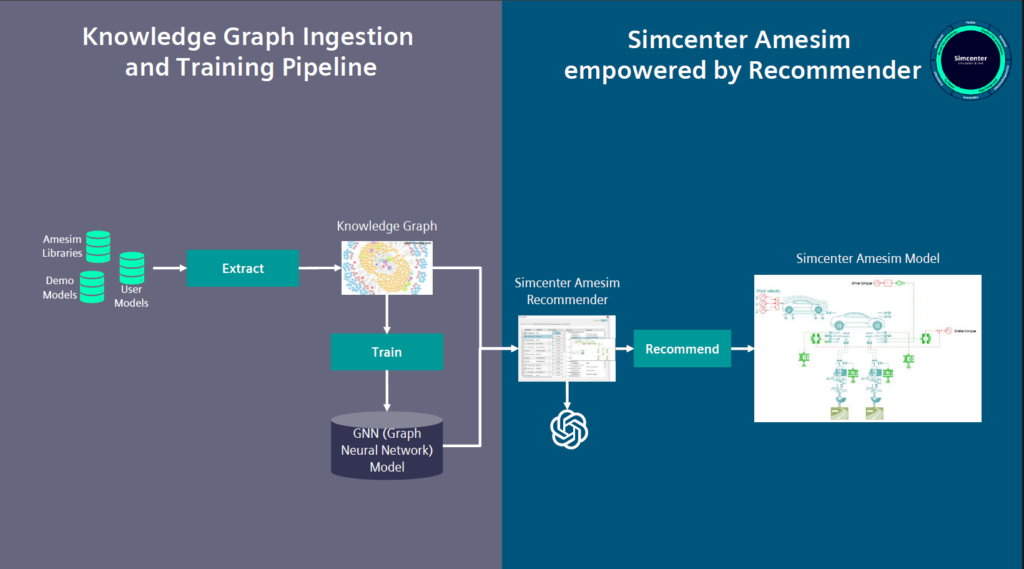
Workflow Assistant
Sketch Creation
Lisa begins to create a draft design aligned with the vision of the architecture team. Her initial challenge is to find a previous design that closely matches what she intends to model. This would allow her to make incremental modifications to meet the new requirements. Thankfully, with the help of the Recommender’s Chat interface powered by ChatGPT, she can quickly discover the most relevant design that utilized the same key components from her requirement list within a minute. As she hovers her mouse over the unconnected components in the sketch, the Recommender continuously suggests the next component in the design. This eliminates the need for her to search through unfamiliar libraries. After conducting a thorough comparison, Lisa successfully creates a sketch that adheres to the given architecture. This marks an excellent first step in her journey.
Submodel Configuration
Lisa realizes that she needs to explore alternative combinations of submodel for the design, even though some components already have assigned submodels. However, manually removing all the existing submodels and selecting new ones for each component would be extremely time-consuming. Moreover, choosing the appropriate submodels requires expert knowledge. Fortunately, when Lisa switches to the submodel mode, she discovers that the Recommender can suggest multiple combinations of submodels with varying confidence levels. These suggestions are generated based on patterns learned from past projects. Lisa decides to remove several submodels that she wants to further explore and relies on the Recommender to propose suitable combinations. With just a single click, the suggested submodels are assigned to all the selected components. Lisa carefully reviews these suggestions and makes minor adjustments as necessary. In the end, she successfully obtains a model architecture that aligns with the specified requirements. She feels confident to proceed to the next step in the workflow.
Parameter Configuration
Lisa is faced with the daunting task of assigning hundreds of parameters to the components in order to meet her requirements. She has heard from the Simcenter Amesim experts at X-Car that typically 80% of the modeling time is spent on testing different parameters. The modeling team has cultivated a trial-and-error culture as part of their daily work. However, Lisa is confident that she can seek assistance from the Recommender for this task as well.
To her delight, she discovers that the Recommender provides a chat interface where she can directly ask questions about the parameters of the currently selected component. Additionally, the Recommender displays an overview of all parameters that violate the learned best practices. When exploring these notable parameters, the Recommender offers detailed suggestions for suitable values and presents a statistical distribution of recommended values. Lisa tries out the recommended parameters and compares them with the architectural parameters specified in the project. After making some adjustments, the Recommender confirms that all the parameters adhere to best practices. With the green light from the Recommender, indicating that she can proceed with running the simulation to obtain results, Lisa feels confident and prepared to move forward.
Simulation
Lisa proceeds to run the first simulation of her car model. During the simulation, she plots various variables to assess performance data. To her delight, she successfully obtains a velocity plot with the predefined load on the chassis. After consulting with the architecture team, she realizes that the initial result is relatively reasonable, although some adjustments are still necessary and certain parameter ranges need further exploration and testing.
The experts emphasize that without the assistance of the Recommender, achieving this result would have taken at least one week for a beginner like Lisa. She appreciates the time-saving and guidance provided by the Recommender, which enabled her to make progress more efficiently.
Lisa’s Next Steps
As Lisa becomes more proficient in using Simcenter Amesim and her model becomes stable, there are still valuable actions she can take with the assistance of the Simcenter Amesim Recommender. Once the architecture team has signed off on the design, Lisa can consider the following steps to leverage the full potential of the Simcenter Amesim Recommender:
- Run the ingestion pipeline: By running the ingestion pipeline, Lisa can store her model in the knowledge graph. This allows her to contribute her model to the collective knowledge base and make it accessible for future reference and recommendations.
- Run training to fine-tune the model: Lisa can utilize the Recommender to further train and fine-tune the model, considering the knowledge she has gained throughout the modeling process. This helps the model learn from her experiences and incorporate her domain-specific insights. The fine-tuned model can then provide more accurate and tailored recommendations for future projects.
Conclusion
Lisa’s journey as a new simulation engineer showcases the immense value of the Simcenter Amesim Recommender tool in assisting beginners in effectively utilizing system simulation software. By leveraging cutting-edge technologies such as the knowledge graph, ontology, GNN (Graph Neural Network) and ChatGPT, the Simcenter Amesim Recommender empowers engineers like Lisa to swiftly grasp the intricacies of modeling systems with Simcenter Amesim and accomplish their tasks with greater efficiency.
Internal studies have indicated that this Proof-of-Concept recommender can deliver at least a 50% increase in modeling efficiency. This remarkable tool not only offers progressive learning capabilities, but also provides workflow guidance, a vast training data repository, and support for best practices. These features collectively demonstrate how the Simcenter Amesim Recommender can revolutionize the field of system simulation and enable engineers to thrive in their careers.
With the Simcenter Amesim Recommender, simulation engineers can confidently tackle increasingly complex projects, knowing they have a reliable and knowledgeable assistant by their side. This technology ensures that simulation engineers remain at the forefront of their field, equipped with the necessary skills and expertise to overcome challenges, and achieve remarkable results.
- Lisa is a fictional character who is an inexperienced simulation engineer.
- X-Car is a fictional automotive company that serves as the context for Lisa’s simulation project.
- A submodel is a behavioral model associated with a component in Simcenter Amesim.
- Simcenter Amesim Recommender is a Proof-of-Concept app designed specifically for Simcenter Amesim, aimed at assisting engineers in the modeling process.
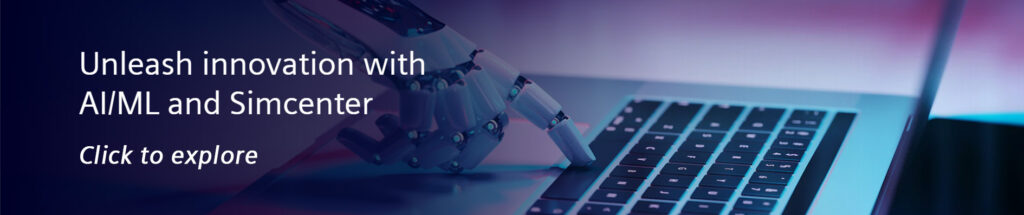