On the ongoing evolution of industrial simulation
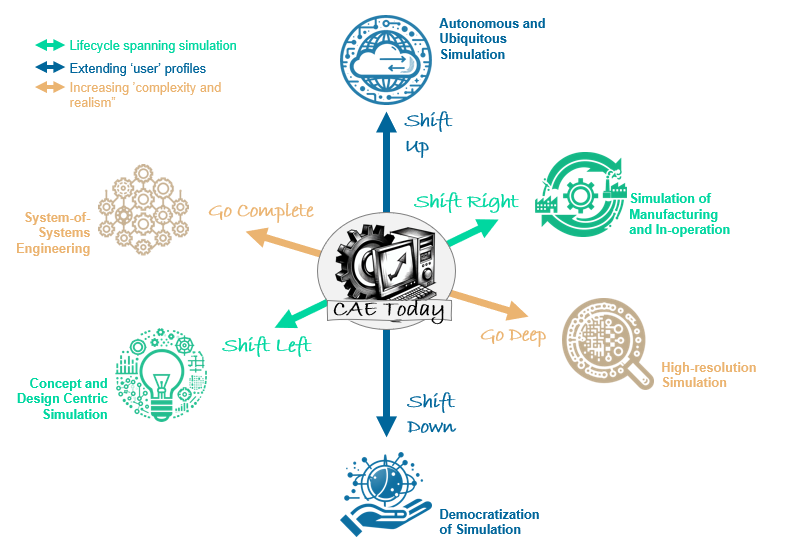
Industrial simulation technologies are a foundational tool for industrial innovation, as well as a key enabler to help solve the grand challenges facing humanity. They are an indispensable part of any product development process today. Hardly any industrial product is not being simulated to some extent during its development. Simulation is already a strategic technology for any company, but its importance will only increase further. Despite this trend, simulation as of today is still only accessible by a very expert centric user group. Technical as well as non-technical barriers prevent adoption of simulation by anyone and all who might benefit from it[1].
Historically, simulations were constrained by the availability of computer hardware resources. Currently, we stand at a pivotal juncture. Due to the exponential growth in computational software and hardware capabilities, the industry has reached a critical tipping point over the past few years. Computations that took a week back in 1979 on the first personal computer (IBM 5150) can now be completed in 3 milliseconds on a MacBook Pro[2] – a speedup by a factor of 272 million in 44 years. On top, many simulations benefit from an additional similar factor in terms of speedup due to advancements in the simulation software itself[3].
“We have transitioned from a paradigm of computational limitations, where the access to appropriate compute resources was a major hurdle (and manual efforts were negligible), to one where human expertise is becoming the scarce commodity. Accessibility to experts being able to operate complex simulation software is now becoming a bottleneck.“
So where next for industrial simulation?
Although not untrue – to say that Computational Aided Engineering (CAE) is still a niche activity, only for the benefit of those expert enough to employ it, does not reflect the growth of adoption of CAE over recent years. Yet still simulation enabled innovation has not reached its full potential. To evolve the adoption of simulation for any and all who might benefit from it, the following 6 possible vectors offer promise in taking industrial simulation from where it is today, to where it might be in the future:
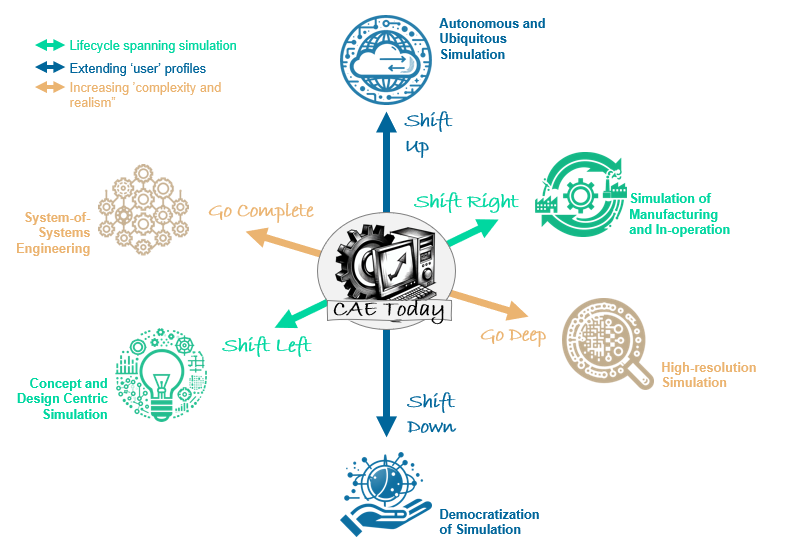
Although ‘shift-left’ has gained traction in the CAE market of late, these are further ways in which industrial simulation will advance. They can be classified as follows:
- Extending User Profiles: This shift in users covers not only democratizing its use [Shift Down] but also enabling the consumption by fully autonomous systems, e.g., in the context of Generative Engineering integrated as background capabilities via API interfaces and micro-services [Shift Up].
- Lifecycle-Spanning Simulation: This includes using it earlier in concept discussions [Shift Left] to the use of simulation during manufacturing, operation, and service [Shift Right].
- Extending Use Cases: This trend of driving the expansion of simulation technology includes expanding the scope of simulation from addressing higher complexities on a system level [Go Complete] to going deeper in the addressed physics [Go Deep].
“Evolve the adoption of simulation for any and all who might benefit from it.”
Shift Left – concept and design centric tools
A ‘Shift left’, or ‘frontloading’, approach entails the application of simulation, not as a late-stage verification tool, but as a way of de-risking early-stage design ideations and concept go/no-go decisions.
“Make mistakes early and often, just don’t make the same mistake twice.“
Whereas today simulation tools are commonly operated by dedicated CAE engineers, the shift left trend addresses CAD engineers, designers, or even sales practitioners. For example, while there are a few million CAE engineers, the number of designers is estimated to be an order of magnitude larger[4].
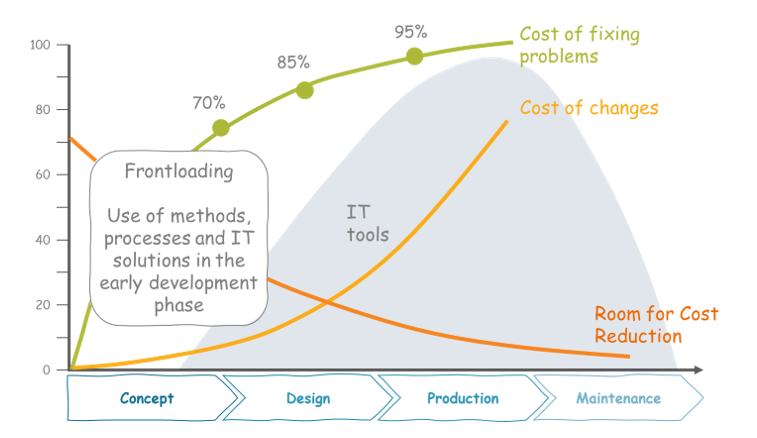
To get there, CAD engineers and designers still require the ability to simulate all the relevant physics of their design but will not suffer the expertise and associated software cost required to actively curate a simulation model[6]. The simulation needs to work ‘out of the box’, repeatedly and dependably. They need to be (self-)explainable providing an understanding and the level of confidence that they can be used for taking engineering decisions. The desire is to have each and every concept variant considered from a simulation perspective by the designer for a first go/no-go determination so that the value of the CAE expert can then be bought to bear only on problematic or challenging design scenarios.
Shift Down – democratization
To shift down the expertise required for application of CAE simulation, it is key to democratize its use to the extent where a much broader range of user personas might benefit from it. This addresses not only the necessity for the Shift Left and Shift Right opportunity, but aims in general to democratize beyond the current scope of user personas that those CAE tools service today.
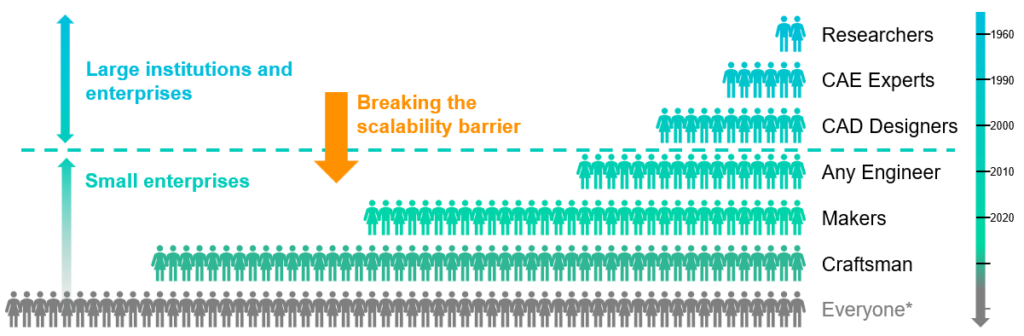
Whether it’s a ‘Maker’ (someone who achieves the entire process, from product inception all the way through to manufacture) as described in this blog by our colleague Matthias Duerr, or craftsmen who, for example, might want to make informed decisions as to where to best place a radiator through to literally everyone who might benefit, even if it is just figuring out how best to dry your hands!
Shift Right – utilization
While the primary use of simulation has historically been during the design and engineering phase, a major opportunity is its utilization during manufacturing and on into asset or process operation. Whilst there are some instances of simulation being used today during manufacturing, with the increasing complexity of production and the individualization of products, opportunities in the use of simulation to support bespoke and emerging manufacturing processes are increasing.
An executable Digital Twin (xDT) creates a live connection between virtual and real worlds. It continuously transforms IoT data through simulation into engineering insights and drives smarter products that can adapt to changes in operating conditions. It can be leveraged by anyone at any point of the product’s lifecycle without the need for heavy simulation software and infrastructure.
The Executable Digital Twin vision[7], in the context of the Industrial Internet of Things (IIoT), will provide the ability to optimize energy usage, prolong the lifetime of products, support prognostic and health management and realize an in-operation remaining useful life (RUL) or life cycle cost (LCC) realtime estimation.
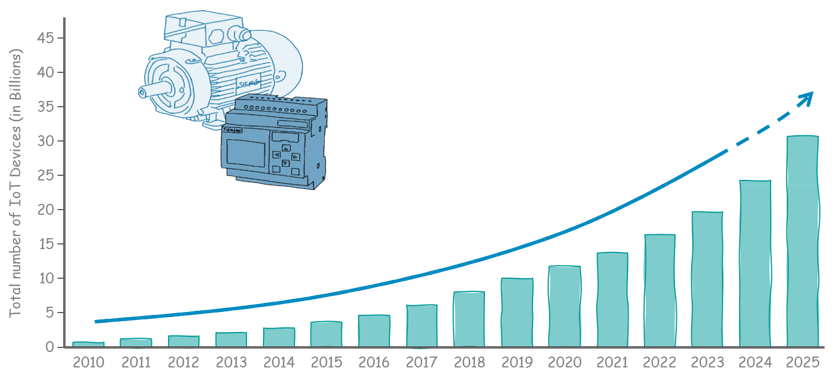
Shift Up – autonomous and ubiquitous simulation
Today simulation is conducted within one or more dedicated CAE tools. That in itself is a limiting factor as to the further adoption of simulation by any and all who might require it. With the emergence of the Industrial Metaverse and Generative AI, physics simulation capabilities might be provided via micro-services that can be invoked from any virtual environment, or might be constantly running so as to provide an ongoing physical behavioral update to the virtual environment and all assets within it.
Shift Up would herald a ubiquity in physics simulation, happening all the while, in the background, without requiring human intervention to massage or coerce a simulation model to be able to provide output. With such automation, physics simulation could be considered by any virtual environment, in much the same way as optical simulation (i.e. ‘graphics rendering’) is done today.
Go Deep – high resolution subscale simulation
CAE roadmaps have provided a significant enhancement in simulation fidelity and multi-physics capabilities in recent years, including an increased capacity to resolve complex geometric details and more intricate physics interactions. Continuing the quest for more realism towards truly realistic representations is at the heart of increasing the capability of CAE tools for more tightly integrated multi-physics simulations. However, the growing complexity of systems, along with the ongoing pursuit of increases in efficiencies and reductions in overdesign margins, furthermore necessitates the optimization of physical behaviors across an even broader and deeper length scale spectrum than today.
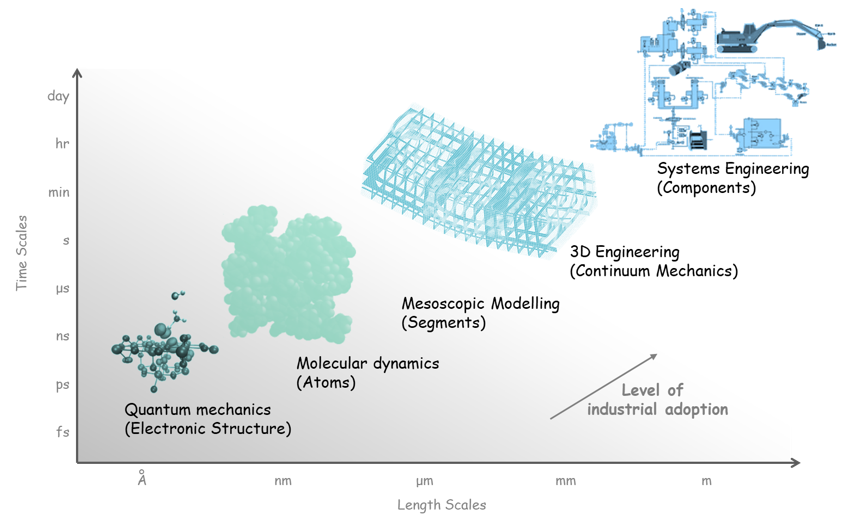
Current engineering simulations often face limitations, typically only considering a restricted array of physical phenomena or constrained in the range of length scales they can address concurrently and with a continuum assumption. Designing at even finer scales however opens the door to leveraging structures that were previously overlooked. A general example of this is in the field of advanced material design. Here, cutting-edge technologies allow for the manipulation of material characteristics almost at the molecular level. Such precision in material design can lead to innovative applications in various fields, including new approaches to chemistry and material sciences. Despite early steps[9], this area remains largely unexplored. Similar challenges and requirements are evident in other complex systems, such as batteries, which exhibit intricate rheological and electrochemical behavior. Successfully modeling these complex and nuanced behaviors is crucial for developing next-generation engineered solutions.
Go Complete – systems-of-systems engineering
Recently, we have seen increasing complexity in systems and systems-of-systems being simulated and manufactured. This is motivated by miniaturization, tighter integration of mechanics, electronics, and software, and ecosystem thinking requiring the interaction of various actors. Furthermore, autonomous systems, such as robotics and cars, require safe interaction with their dynamic environments and thus inherently the adoption of systems-of-systems thinking. Last but not least, circular economy and sustainability demand lifecycle-spanning approaches, requiring holistic overviews of assets and products. This evolution necessitates expanded simulation capabilities, incorporating all physics and domains be they addressed using 3D or 1D simulation approaches, supporting large-scale systems, mission engineering, autonomous impact analysis, and cross-discipline integration.
CAE tool providers are expanding their portfolios through investments, partnerships, and acquisitions, addressing this need for a more complete ‘systems’ approach, especially of late to de-silo CAx, PLM and EDA. R&D departments must diversify their use of expert simulation tools and increase simulation efficiency, shifting from traditional workflows to collaborative engineering methods to capitalize on the resulting RoI[10]. Implementing Model-Based Systems Engineering (MBSE)[11] and adopting standards like Systems Modeling Language (SysML V2) is but one example of how this might be achieved. With the emergence of the Industrial Metaverse, logically an extension of MBSE, we now see novel and exciting possibilities for systems-of-systems development in photo-realistic, immersive and collaborative environments, bringing the core ideas of MBSE to a completely new level.
The future
The prediction of the physical behavior of products or processes is today a task that is undertaken by dedicated practitioners. A plethora of CAE tools are available to aid and assist them in this endeavor. This landscape will continue to change, just as it has over previous decades.
“Prediction is very difficult, especially if it’s about the future.” (Niels Bohr)
As to how this simulation landscape might change, we have identified 6 vectors along which the adoption and application of physics simulation might evolve and grow beyond the rather ring-fenced constraints it finds itself in today. As to which of these opportunities might be realized first, only time, effort and endeavor might tell.
“Anyone, who has the need, will be able to easily and robustly predict all relevant behavior of individual assets or complex systems up to the required realism and compute time with accuracy guarantees.”
[1] CIMdata (2023): Simulation & Analysis Market Report; Market Analysis Report Series
[2] IBM 5150 (Intel 8088 + Intel 8087 Co-processor, 1979) with 50×103 FLOPS vs. Apple MacBook Pro (M2 Max, 2023) with 13.6×1012 FLOPS
[3] U Rüde, K Willcox, L Curfman McInnes, H De Sterck (2018): Research and Education in Computational Science and Engineering. SIAM Review
[4] M Panthaki (2015): Usability of Simulation Software. COFES 2015
[5] M Eigner (2010): Future PLM – Trends aus Forschung und Praxis. University of Kaiserslautern Blog, 2010.
[6] T Abbey (2017): CAD and FEA User Interface Evolution. Digital Engineering December 2017
[7] H Van der Auweraer, D Hartmann (2022): The Executable Digital Twin: merging the digital and the physics worlds
[8] KL Lueth (2020): State of the IoT 2020: 12 billion IoT connections, surpassing non-IoT for the first time. IOT Analytics
[9] A Merchant, E Cubuk (2023): Millions of new materials discovered with deep learning. Deepmind Blog
A Merchant, S Batzner, SS Schoenholz, M Aykol, G Cheon, ED Cubuk (2023): Scaling deep learning for materials discovery. Nature
[10] EC Honour (2013): Systems Engineering Return on Investment
[11] INCOSE (2023): Systems Engineering Handbook: A Guide for System Life Cycle Processes and Activities