Going beyond automation with the Industrial Copilot – Part 2 Transcript
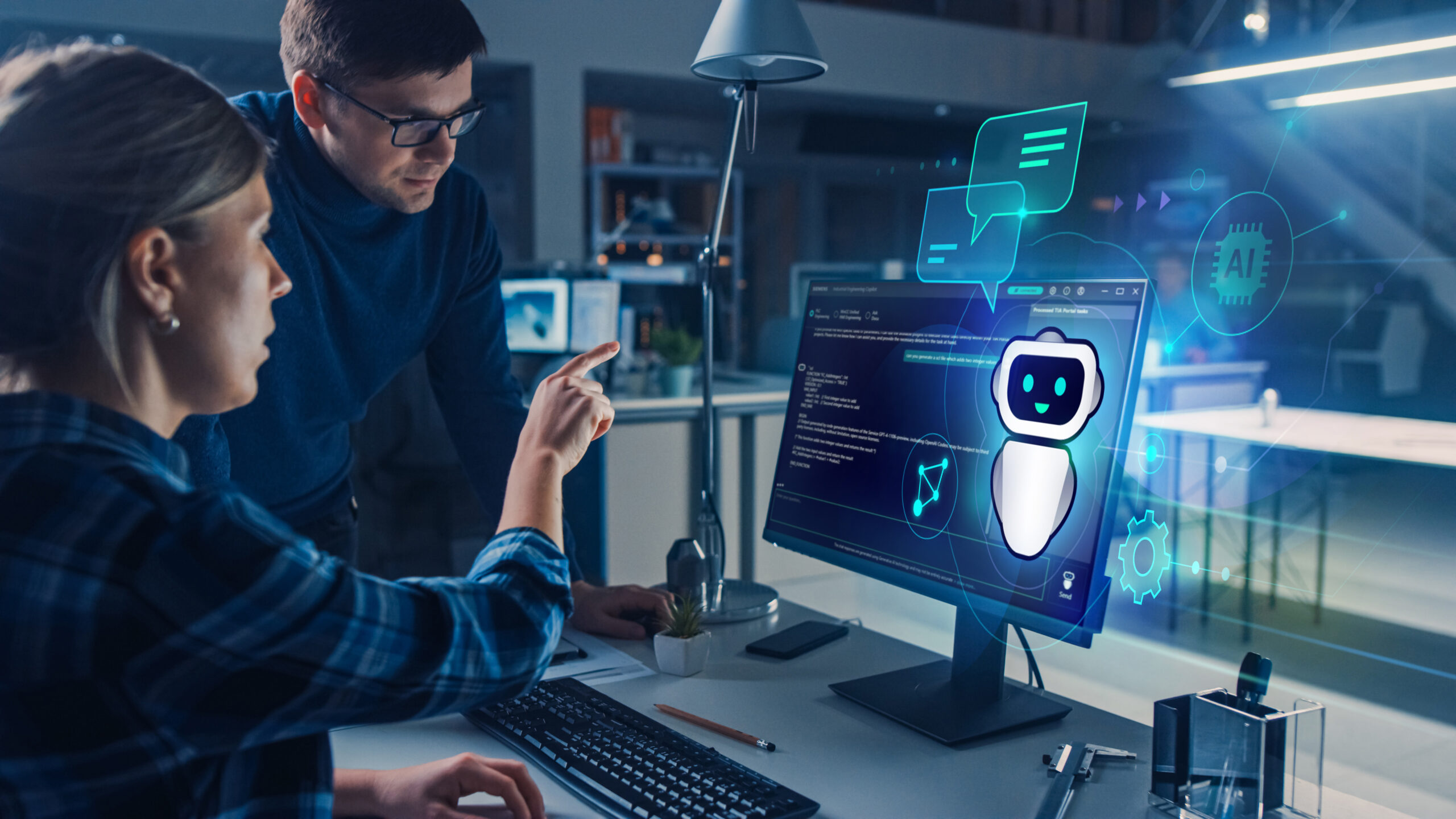
Integrating AI across a broad industrial ecosystem has both many challenges and benefits. In a recent podcast, guests Michi Lebacher and Alessia Bortolotti discuss some of the considerations of bringing the Industrial Copilot to every level of the Siemens software ecosystem. Check out the full episode here or keep reading for the transcript.
Spencer Acain:
Hello, and welcome to the AI Spectrum Podcast. I’m your host, Spencer Acain. In this series, we explore a wide range of AI topics from all across Siemens and how they apply to different technologies. Today, I am once again joined by Michi Lebacher, head of AI and digital business at Siemens, and Alessia Bortolotti, customer discovery manager at Siemens AG to discuss the applications of AI and industrial copilot.
In the previous part of this discussion, we ended things talking about the entire ecosystem of Siemens products that are being supported by the industrial copilot and integrating with AI. I think it would be a great place to pick up here today and starting with looking at some of those benefits you’re already seeing by this broad-reaching integration across an entire ecosystem of products and tools and bringing AI to that system, as it were.
Michi Lebacher:
That’s obviously a very important question. My motivation and our motivation for doing that is of course that in the end we develop something that brings real value and benefits to our customers. Not so easy to answer because the value chain is quite extensive, but of course I can give examples for those use cases where I’m quite close. So coming from the factory automation part of Siemens, it’s especially kind of like the industrial copilot for engineering. Here the idea is everyone who lives in the IT space, so to say, is now used to have some kind of a GitHub copilot that has some coding. And we want to transfer exactly that logic to the world of automation engineers, because we have an engineering tool, which is the TIA portal. And with the copilot for engineering, we already see this benefits on many different layers.
So the first layer is it becomes more convenient for the user. So they more easily can generate PLC code. It’s easier for them to generate HMI screens. So that’s helpful, but it also automates away repetitive steps. So all these things where you need to write and write and write again the same stuff. Now this is much faster. You have huge efficiency gains there. And on top of that, and I think also that’s a huge value of the copilots, you can go from beginner to expert very fast. So it’s not only a tool that you as an experienced automation engineer want to use to become more efficient, but it’s also super helpful in order to onboard new employees.
So really just beginner to expert, and this is something we hear everywhere. It’s not only in the case of do you have enough experts that do the PLC programming, which is a complicated task, right? So a PLC is a piece of automation equipment that is in the end the heart of all automation systems and you need to program it. So it’s complicated and therefore you are desperate in search for these experts. But it’s everywhere. So it’s also in case of operations. So for example, a huge problem in operations is typically downtime. This is the thing that’s most crucial. At the moment where the red light is going on and your machine halts, you’re really in trouble because when every minute counts and every minute is worth hundreds of thousands if not millions.
And also here you need to have a lot of very experienced people. And if you have a system that A, makes them more efficient in resolving machine issues, you can save a lot of money. And the other thing is, if you have a system that makes it easier for people that have less experience with that, you really have a huge lever. And we see that. We did hundreds of interviews and interactions with customers. This kind of value proposition, it sticks, they like it and they definitely say, yes, we need to have it. And now also because you specifically asked, do we already see the benefits? The nice thing is yes, we already see them materialize. For sure, for the copilot for engineering. So this is quite mature by now. But also for the copilot for operations. We do that together with our own factories of course. So we drink our own champagne, so to say. And there we already see it’s in heavy usage. They like it. And we do it with pilot customers where we also get great feedback. So I think we can deliver and live up to this value proposition.
Plus, we have one big flagship partner. It’s not only our only partner or customer, but Scheffler kind of like is a reference we can give also publicly. So Scheffler also goes to fairs with us and kind of like the biggest proof that Scheffler came to us and said, “Okay, this is super interesting. This engineering copilot is for sure something we need. And please also, let’s work together on the operations copilot. Let’s develop that together.” So it’s not only that we at Siemens believe that we see benefits or it may be our own plans, the benefit, but we see this market pool. And I would say this is a huge opportunity for us and the benefits are already there.
Nevertheless, it’s important for me also to make clear we are right at the beginning of everything. So no one says that the capabilities that we have with gen AI right now are already perfect. So I always think about it in terms of an S curve. So in the beginning, the benefits might be smaller, but then suddenly with all the innovations that we see, the curve gets steeper and steeper and we get more and more. And I would say we are on a good way to that.
Of course, this also comes with the hurdles, I guess anyhow, we talk a bit about hurdles. But there’s of course also a price to pay for these benefits. You need to be open as a customer for generative AI. So there are still people that are conservative with respect to that. But my feeling is the more we show the value, the clearer it really becomes that there is something and that also convinces customers. And on top of it, speaking of benefits, it’s of course important also to mention a bit. Many companies are experimenting with gen AI so far, but the benefits that it brings to do it together with Siemens now from a customer perspective is that we can leverage a lot of unique selling points that Siemens already has.
So, we are very good with automation equipment. We are a market leader here. We have established tools. So we have the TIA portal or PLC engineering, and therefore it’s a lot of system value if you already have it to have on top of corporate operations embedded there where you want to have it. And the same is when it comes to our PLCs. For the corporate operations, our ideas, we use the dynamic machine data as well as the documents. And since we produce this controllers, we have fast access to this data. So we can leverage a lot of system value that simply comes out of the fact that Siemens has so much experience with this kind of domain. So this is also something which we also get back from the customers that it’s great that exactly Siemens is doing that.
That was my outline on, do we already see benefits? And I would say it spans wide from we see benefits, but I think much more to come in the future. I don’t know, have I missed something, Alessia Bortolotti?
Alessia Bortolotti:
Actually I think you explained everything here. Maybe one small comment from my side. You talked about the benefits. You mentioned also some challenges or some reluctances from the customers, but here we are really trying to be customer-centric, to talk to the customer before starting really testing with them, the copilot, but also through the old piloting phase, for example. So there we are trying to collect all these feedbacks, all the maybe challenges that they are facing, but also we are trying to validate what we think is a benefit and see if this really fulfills their needs. Just to highlight this customer perspective.
Michi Lebacher:
Excellent. Excellent, that one.
Spencer Acain:
I see. So it sounds like it’s still early days, but you’re already seeing some very real, very tangible benefits to deploying AI on top of a lot of our existing Siemens products that many customers are already relying on and just sliding it in there as an extra layer that brings an extra value on top of what companies were already doing and already seeing.
Michi Lebacher:
Exactly. Yeah. Yeah, perfect summary actually.
Spencer Acain:
Oh, great. And you did a fantastic job explaining it then. But it sounds like you really got an AI solution or at least planned for in every area, every section of the market or across all the design planning, factory automation, all of that, all these very different technical areas. So how are you training these models or feeding these models all the data they need so that they can be a good copilot or a good assistant to all these different users across all these different domains?
Michi Lebacher:
Yeah, of course. This is one of the key questions. As already outlined, so to say, the easy part is identifying those tools that we already have where we can stack copilots on top of it in order to create value for the customers. The hard part is how to make it industrially grade. Because as I already outlined in the beginning, we don’t shoot for giving you yet another play around system that you can ask about football and stuff. But for us, it really matters to bring hardened industrial-grade quality to our customers. And this is what they expect from our brand and from Siemens. And therefore, it’s extremely important to find out how, and especially as you asked, with which model and what data you can provide it.
And as usual, the answer is not so simple, especially if you consider the whole value chain. Because if you think about it, the different possibilities are really far-reaching. The first step could be that you say, okay, you create your own foundation model, which really would be go and collect data for domain where you are extremely good at and create an own model for that. And this is something we are looking into and we are evaluating that. And for sure there is some specific domain knowledge and data that Siemens manages the best. So this is for sure consideration.
What we nevertheless, especially because we also consider time to market to be very important, also consider very heavily is that we work together with established partners in the field. So speaking for the copilot for operations, we are partnering with Microsoft, simply because Microsoft is the market leader when it comes to the provision of cutting edge AI models. So here the strategy would be we take established models and then we either adjust them so that would be the fine-tuning route that you say, okay, you have a model, and then you go into fine-tuning, this is a discussion. Or that you say the more usual pattern that you see now basically everywhere, is that you go for a retrieval augmented generation approach, or RAG approach, that you say, okay, we take the model that we have as a baseline and then we enrich the context, for example, with documents and dynamic machine data and all kinds of complex data structures. And then the secret source is that we tailor the application to be so good that in the end, even with an off the shelf model, you get superior results.
In principle, we investigate all of that and we look into all of that. I personally have the feeling this comes in waves. So in the beginning, the RAG approach makes a lot of sense and also combines very well with our partner, Microsoft. But in the future we will see different variants. We will see fine-tuning and maybe even old foundation model. So it really depends also on what do you want to deliver, on what kind of quality do you need to deliver it, and lastly also what in the end you want to achieve.
So for example, fine-tuning makes a lot of sense if you serve many customers with one big model or also a whole foundation model. But if you want to say, okay, for many different customers you would need different models, then potentially the RAG approach is better. So it really depends a bit. And we are in the exploration phase at the moment. So in the end, I would say we already can achieve a lot with these RAG applications. So this is what we see in the copilot for operations where we at the moment strongly investigate RAG and also test that together with pilot customers.
I have the feeling that customers love the situation that I go to them and say, okay, I have something that works the very moment where you give me your machine context, for example, documents about your machine, as well as access to your machine data, and then my copilot for operations already provides value to you. In comparison to, okay, let’s first collect data for a year, then we fine tune a model and then you can see something. So this is the beauty of the RAG approach that I can deliver the value almost instantaneously. So that’s very, very nice.
Yes. So kind of like the question super valid that you asked, I would say depends on the application, depends on the level of quality that you want or must achieve. And we are on the way to exploring all of that. But of course, it’s not only a question of the models, but it’s also a question of the data. So even if we would decide that we create our own models and fine-tune models, it also must be in accordance with our customers. In the end, it’s their data. And of course you can make respective contracts that you are allowed to work on that data, but this is something to be found out together with our customers. So the least thing that we want is stealing customer data or making this appearing.
So it’s really for us, we want to be recognized as a trustworthy partner in the AI space here that does not sell the data of the customer, that does not misuse it, and therefore we really take, especially the data part, extremely serious.
Alessia Bortolotti:
And maybe to add on that, to highlight again this customer’s perspective, the customer view as you were saying. So we are currently evaluating that with the customer because we know that for some of them, for example, fine-tuning is completely fine. They don’t have any problem with that. They know that giving data or sharing more data means also then to get maybe a better model, but at the same time, some other customers won’t accept it, again for data privacy reasons. So they have maybe data sensitive domains, and they are feeling really not so confident in sharing that.
When then talking about, for example, specifically the data formats. Here we have also a wide range of data that customers expect us to consume. So here specifically for the copilot for operations, this ranges from PLC data over documentation as PDF, for example, up to even SAP systems. And even when thinking about a more broader context here, the data modality range is even bigger, for example, with CAD data and so on. So here, I think really talking to the customer, seeing what they need, try to fit this needs is the best way to go. Yeah, for sure.
Spencer Acain:
Sounds like there are a lot of challenges when it comes to bringing these type of AI tools to customers and to end users and everything like that. And you’re never going to have just a simple one size fits all or one-stop shop solution that fits every person for every need for every application. But at the same time, it sounds like you took that into account and you’re really building a comprehensive suite of tools here that can meet all of these varying requirements.
And I think that is a great place to end this episode. I’d like to thank Michi and Alessia for joining me today. And once again, I have been your host, Spencer Acain, on the AI Spectrum Podcast. Tune in again next time as we continue this conversation on the exciting topic of AI and the industrial co-pilot.
Siemens Digital Industries Software helps organizations of all sizes digitally transform using software, hardware and services from the Siemens Xcelerator business platform. Siemens’ software and the comprehensive digital twin enable companies to optimize their design, engineering and manufacturing processes to turn today’s ideas into the sustainable products of the future. From chips to entire systems, from product to process, across all industries. Siemens Digital Industries Software – Accelerating transformation.