Riches to RAGs: Understanding Retrieval Augmented Generation
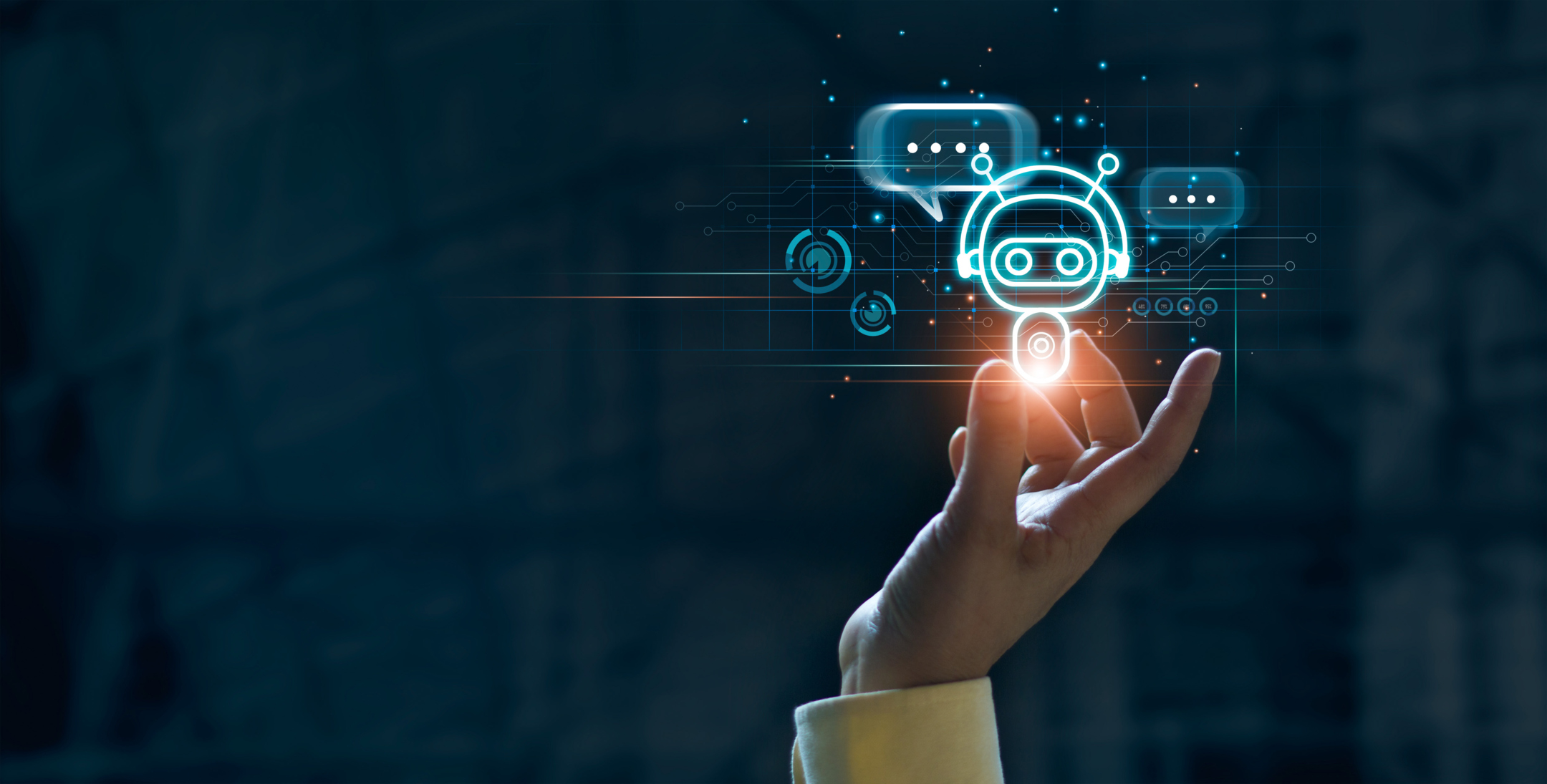
Generative AI is a hot topic across every industry right now but, as the initial hype begins to fade, the applications where the technology can truly shine are starting to come to light. One of those areas is retrieval augmented generation, commonly known as RAG, which helps transform generative AI from a chatbot to an interactive database of knowledge spanning any and every subject imaginable. Combining RAG with the extensive documentation and design data common to the worlds of manufacturing and design and its easy to see why this is one of the technologies that has leapt to the forefront of AI research in industry.
RAG: More then a smarter search engine
RAG isn’t as simple as just asking ChatGPT to google something and summarize the results but rather requires that all the relevant data be analyzed and sorted into a relational database ahead of time in a process called embedding. Creating these embeddings still relies on an AI model however, in this case a specially-designed transformer model that can “understand” the importance of various words and phrases in the overall meaning of a document and then numerically represent that information.
Embeddings, along with chunking, a process by which a document is divided into small, but meaningful, segments or “chunks” are at the heart of what makes a RAG system tick. By applying the same process to a search query, a system can use various numerical methods to compare the input, in this case the query, against all the stored information in the relational database before ranking the results by relevance. From here, the resulting selections of most relevant information can be fed to a generative AI model which apply its advanced natural language processing abilities to synthesize the information into an easy to understand, human-readable form.
Applying RAG to a large body of information not only makes the information more accessible, since it can be searched through by content rather than exact keywords, but also creates a smarter and more reliable AI system as well. By backing up a generative AI model with the hard facts provided by a RAG system, the AI becomes less likely to hallucinate and provide garbage answers, instead providing a conversational interface to a potentially vast storehouse of knowledge.
AI builds a web of knowledge
The foundation on which any RAG system is built is raw data, something the manufacturing and design industries has in great quantities, a fact that has always been as much a blessing as a curse. Years of past designs, manuals, tests and other documentation can be considered one of the great assets for any company but equally so, finding pertinent information for a particular situation or examining the data for trends at large is a daunting task.
Applying RAG to this vast data set not only makes it more accessible via content-aware natural language search, but also increases the value of the data itself. Now, thanks to ease of accessibility, the valuable knowledge stored within years of design data is at the fingertips of anyone who needs it, helping to better preserve and pass on that knowledge in a new generation of engineers and technicians.
Building an RAG system can also serve as the starting point for applying AI to existing data in new ways. With an existing relational database, new AI analytical solutions can be more easily developed and implemented, offering the potential for greater insight all the way from top floor to shop floor. Leveraging AI can extract new value from existing data by looking at datasets holistically and presenting the results in a human readable way, a next step forward from what RAG can offer by developing insights rather than just retrieving information.
In an age of information, it should come as no surprise that a system that makes using and accessing information easier would be among the hottest topics of interest for massive companies all across the globe. As RAG and AI systems as a whole continue to develop, they will reshape the way humankind as a whole interacts with the centuries of knowledge our civilization has produced, leading to new insights and a broader scope of knowledge for all.
Siemens Digital Industries Software helps organizations of all sizes digitally transform using software, hardware and services from the Siemens Xcelerator business platform. Siemens’ software and the comprehensive digital twin enable companies to optimize their design, engineering and manufacturing processes to turn today’s ideas into the sustainable products of the future. From chips to entire systems, from product to process, across all industries. Siemens Digital Industries Software – Accelerating transformation.