Exploring Generative AI Part 3 – Transcript
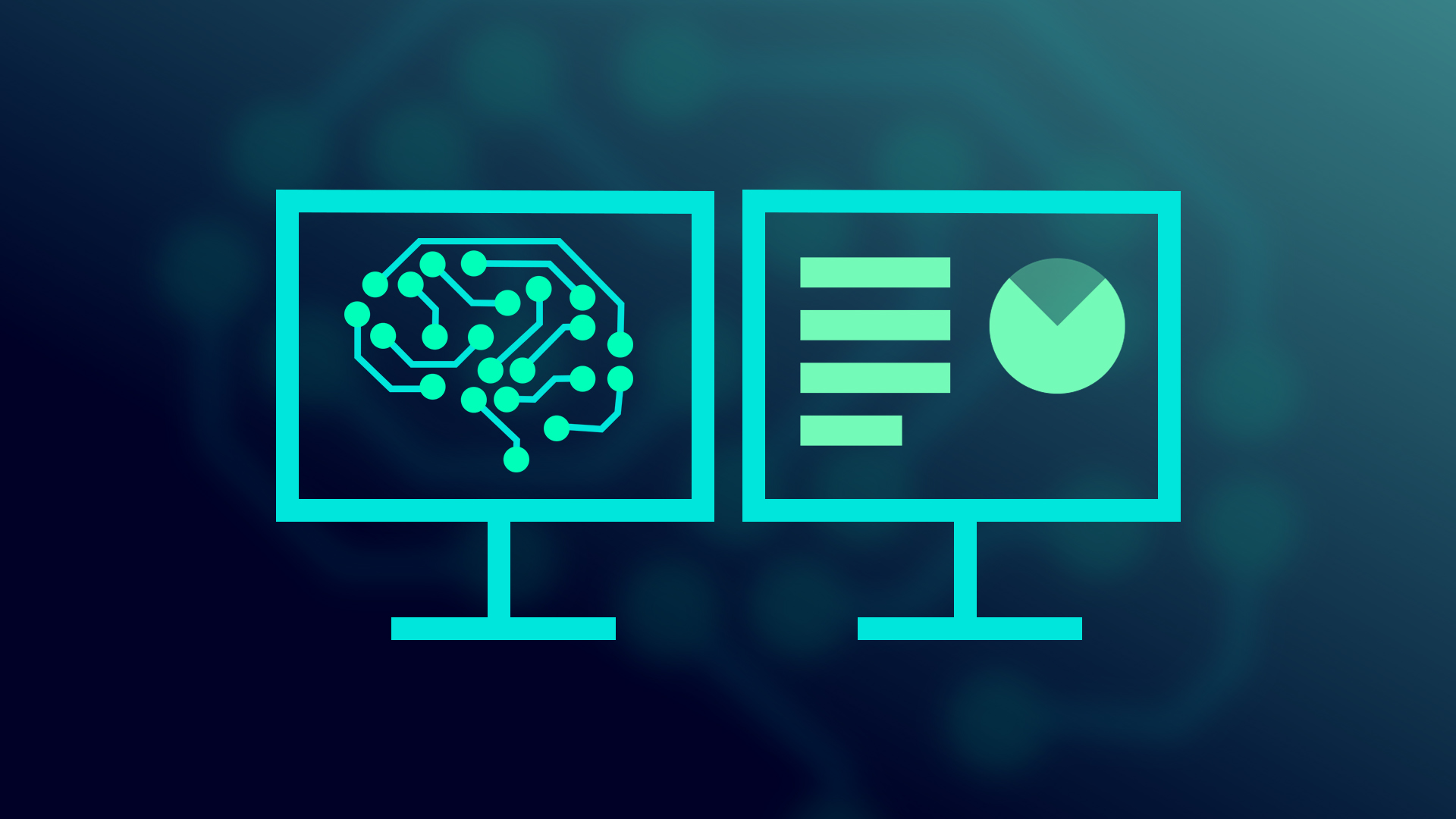
In a recent podcast, available here, I continued a conversation with Dr. Justin Hodges about the ways generative AI will change the way people and technology interact, and what that means from the perspective of trust.
Spencer Acain: Hello, and welcome to the AI Spectrum podcast. I’m your host, Spencer Acain. In this series, we talk to experts from all across Siemens about artificial intelligence and how it does apply to various technologies. Today I’m once again joined by Dr. Justin Hodges, an AI/ML technical specialist and product manager for Simcenter to discuss the many ways that generative AI is reshaping the world of engineering and simulation. So I think kind of building on the idea of AI connecting multiple areas and really to build more functionality, I think … So you’ve mentioned kind of the idea of AI generating geometries or meshes or CAD models, and that kind of reminds me of an example I once saw of generative AI model that took just from a simple single 2D picture of a car, it generated a complete functional driving mesh like in a 3D animation software. So of a 3D car that could drive with lights, turning wheels, all of that kind of stuff. So obviously, it’s kind of a simplistic example, but going forward, how do you see AI being able to do this from a very simple input of that picture going all the way to a fully functional mesh or in the future, like a fully functional design, if it saw a picture of a car, it could know to create the engine, the transmission, the steering system, and all of that, and just generate fully functional 3D CAD and models and all of those sort of functionality within the model itself?
Dr. Justin Hodges: Yeah. So not to oversimplify, but we could put some users in the category of sitting at their computer, designing something and we could put another category of users into operators. And I think that in the operator case, you may be inspecting something, running a physical test, monitoring performance of a fleet or a certain set of equipment. And in that case, it would be really helpful to even have these directional quick and dirty conversions of things like images to models, stuff like that. And I think it’ll really make them more productive as they can, for example, take pictures of things and then have a machine learning model tell them this is working properly or this is not working properly, or just basically as well linking things they probably don’t have time to do. Maybe if you run a water treatment plan or a food processing sort of heavy equipment, maybe you realize, okay, tomorrow I’m going to change to this configuration. I’d love to run a simulation on it, but maybe that takes a full day. I just don’t have time to operate and do my job. So I’m just going off of past experience that when I switch configurations, maybe I switch the impellers or the RPM and the speed of rotation, I just have a general basis on if that’s safe or not. But what you described means they hit more and more fidelity. Maybe they can just make simple changes in terms of a user interface and it will generate a full 3D of result for them so they can actually afford to get a full simulation result when they make these changes in their operations. So I think in that sense, it’s kind of similar to help me quickly go from a picture of a car to a full 3D thing. And I think that will impact engineers in the design environment as well. But, at least, my first impulse is to talk about operators because those are, I think, people that need answers the fastest and really have the highest limitation on running the simulations and whatnot.
Spencer Acain: So to build on that example of an operator needing a simulation, this kind of ties back in with those models you had because if you’ve completed simulations in the past and now you can just kind of … not only you can generate a model there and then you can kind of feed that into those generated models that could then give you your simulation results like we were talking about earlier. And then you could just kind of make a simple dashboard where somebody who has their main job can just say, Hey, I’m going to make X, Y, Z changes, how will that impact my physical model, and then how will that simulate out, what will happen when I actually put that in and stuff. And they could just have that kind of run for them quickly on the side or with minimal input while they’re completing their main tasks, basically. Is that’s something that you can kind of see?
Dr. Justin Hodges: Then that ties everything nicely. So somebody ahead of time would run simulations, they would make a machine learning model, they would deploy that in a cloud to basically, like I just said, have a dashboard that may be no coding, just basically enter some few numbers in, have a slider bar, and then automatically when people are on the shop floor or doing sort of things in a plant, they can have a tablet, it can connect to the same dashboard on the cloud that the user set up for them. And then they can enter data in and numbers in for how things are performing on the floor in real life as they walk around. And it would basically call on machine learning model trained on real simulation data that is trustworthy and high fidelity. And it would just tell them in a fraction of a second what the resulting changes may be, or more information about the behavior that’s going on for the limited amount of dimensions and data that they get from just monitoring the equipment and maybe the readings on meters and stuff like that. And that’s really a strong case there where AI gives them more information.
Spencer Acain: Yeah, I mean, that’s all … It really sounds kind of out there right now, but it’s incredible that we’re actually seeing this kind of becoming a reality. Even five or 10 years ago, I don’t think anybody would’ve thought that this would be possible. But at the same time, I think a lot of the applications we’ve talked about today have been kind of critical. So if whatever results you’re generating for a car or maybe for in a factory, it’s like if these generated results are wrong or there’s a small error in them, they could have some serious ramifications. And I think in the context of generative AI, I am pretty sure we’ve all heard of the stories of ChatGPT, just kind of very confidently throwing out an extremely wrong answer. And it says the year is 2238 or something. It’s not for 200 more years, it’s not. And so how do you account for that in these kind of very critical applications where millions or billions of dollars could be on the line, or even people’s lives could be potentially in danger if the AI is wrong about something or has one of these hallucinations?
Dr. Justin Hodges: Yeah, that’s a huge society-wide effort, I would say, to try to mitigate some of those scenarios and outcomes that are really poor. There’s probably some tenets there that you can say would help a lot. Trustworthiness and explainability. You could say bias and fairness. You could just throw out control and safety for how the models are used probably as well, uncertainty. So at least there’s some metric there for confidence and things like that for the user to have alongside the predictions. And it’s on all of us to become more educated and create more guidelines. So I think those are some tenets that are really key to ensure that we use it responsibly and we can trust the results. And then to put a bit more detail to it, maybe I’ll talk about explainability because I think that one’s particularly exciting and it’s important because the very first thing when you said define generative AI, I said, these are inherently very complex models, and they arrive at pretty specific statements, outcomes, stuff that they generate. So it’s really important that we try to make them as explainable as possible, meaning that it brings to light why it made the decisions and that it did, and the decision-making process. And this is similar to other approaches where you focus on attention mechanisms or activation visualizations or some key terms there in that area. But basically, which part of the data has the largest influence on the model, which type of features or inputs are the most heavily weighted towards the outputs on why they do what they do. So I mean, there’s various approaches in there to just provide that basic level of understanding. Bias and fairness. I mean, that was the other one I think I mentioned. It comes down a lot to other ones you see in ADAS and computer vision. Is the data that you provided well-rounded, is it fair to all types of people, types of weather, types of road conditions, types of car conditions, I mean, it’s really the responsibility that people train the models to make sure that bias is really mitigated or at least documented and understood. And then lastly, I think I’ll just skip the middle few and go to education and guidelines. As a society, we’re slowly making strides to understand what they can and can’t do. I think now you could show people probably a question in three different answers, and they could probably spot the one from a bad old GPT model and probably one of them that’s probably a high-fidelity GPT model and maybe even the human response depending on the prompt. So I think that’s a silly example, but I mean, I think that more and more we’re learning about … we’re educating ourselves on these models and the types of things they’re good at and the type of things that they’re not. So yeah, part of the application, when the people use the model, is also … it’s also on them and the provider to make sure they’re equipped, educated with guidelines, et cetera. And then in terms of CAE and engineering, I mean, we’ll always try not to throw out traditional ways of doing things that are conservation laws, physics, things that have worked for many, many years that we know are trustworthy, things like that. I mean, no one’s trying to replace anything. So I think the more physics-centric physics-aware, basically just posing the problem that you’re trying to apply machine learning to in a conceptual and physics-based way, that way you’re using the model as a means to an end, not as the authority as to what’s correct and what’s not. So I rambled a bit there, but I think those are some of the key things that come to my mind when we say what’s important for generative AI in terms of how we trust it, how it can be used in a non-harmful way, et cetera.
Spencer Acain: I think that was actually a great answer. It sounds like it’s going to be a very nuanced and long discussion on trustworthiness, fairness, and education. At the end of the day, it almost sounds like it’s a tool created by humans for human use. So we as the human operators of the tool will need to be educated and ready to use it and prepared on how to use it just like we would for any other tool. As a simplicity example, it’s like you can’t be expected to operate something like a heavy construction machinery without training. It could be very dangerous. And in a sense, AI is almost the same way. It’s a very, very powerful tool, and you could do a lot with it, but you have to be ready to use it, be prepared to use it, and understand what it is and isn’t capable of doing.
Dr. Justin Hodges: Yeah, and I think that in different organizations, I mean, people tend to contribute from different roles, and I think that this will help it be sort of just one itemized thing to supplement. And I hope most organizations are not just unilaterally supplementing everything with or changing or replacing everything with AI because obviously, it has appealing and attractive use cases, but you need to keep it in check.
Spencer Acain: Yeah, absolutely. I think that’s a fantastic way to encapsulate this. And before we wrap this up at all, is there anything else that you’d like to add or some interesting topics, or anything you want to share before we close this out?
Dr. Justin Hodges: Maybe just a call, a call to get your hands dirty and to try stuff. I think the majority of the people I talk to would be new to AI and machine learning as a practitioner, but just don’t be discouraged. There’s so many avenues and free tools out there today. I mean, my go-to sort of recipe for how you can learn is go on Coursera and take the machine learning specialization. It’s like three classes, but it’ll pretty much give you a concept overview of a lot of things I think is really good. You can get free compute resources, so you don’t even have to have a GPU or anything fancy as far as hardware, just a computer to access the internet, make a Google Colab account, make a Kaggle account, whatever. They both have some free compute time. And what’s funny is even in those tools, I mean, you should know Python to be able to do such things, but as of a few months ago, you can log into Google Colab and right where you type the code, it says “write code.” Next to it, it say … what does it say … something like AI help or generative AI or something like this where basically you can just click over there and then say, please write me a code that can do this, and it will just give you a one-shot sort of answer immediately populated in the field. So the point is there’s plenty of training wheels and sort of water wings to get people going as beginners and not to be discouraged. And I think there’s just so many tools out there to learn that it’s a perfectly good use of time to just tinker around and try different things because we’re just getting offered out there is better and better every day. It’s really amazing this revolution we’re in.
Spencer Acain: Yeah, I love it.
Dr. Justin Hodges: So man, that’s it.
Spencer Acain: That sounds amazing. I’ll definitely be checking that out myself a little later on, I think. So thank you, Justin, for joining me here today, and I’ve been your host, Spencer Acain on the AI Spectrum Podcast. Tune in next time to learn more about the exciting world of AI.
Siemens Digital Industries Software helps organizations of all sizes digitally transform using software, hardware and services from the Siemens Xcelerator business platform. Siemens’ software and the comprehensive digital twin enable companies to optimize their design, engineering and manufacturing processes to turn today’s ideas into the sustainable products of the future. From chips to entire systems, from product to process, across all industries. Siemens Digital Industries Software – Accelerating transformation.