Understanding Engineering Intent with AI Part 3 – Transcript
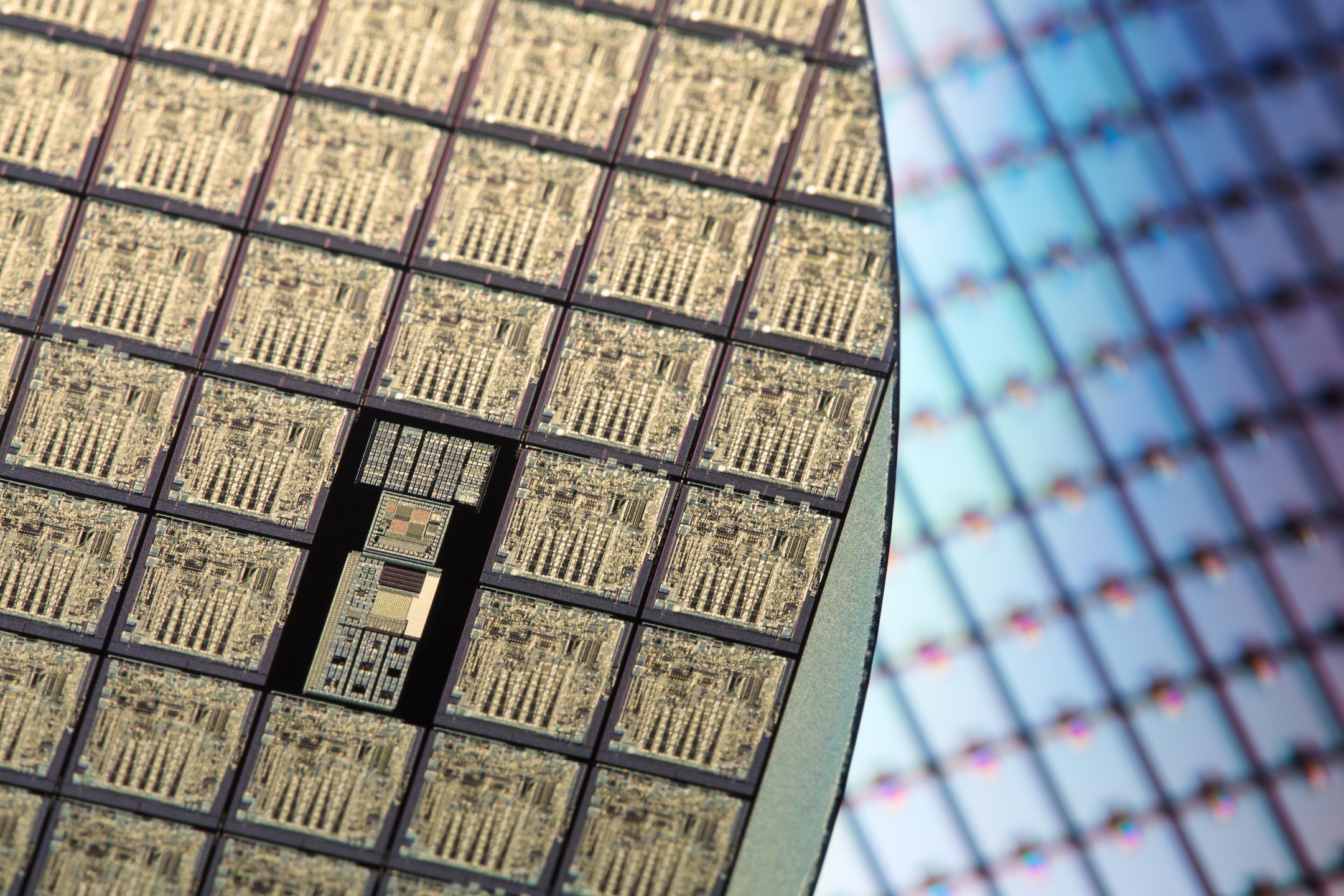
In a recent conversation with Richard Barnett, CMO for Supplyframe we continued an ongoing discussion on understanding engineering intent with AI. In this part, Richard highlighted the value of their training data as well as where he sees this type of AI going in the future. Check out a full transcript below, or click here to listen to part 3.
Spencer Acain:
Hello and welcome to the AI Spectrum podcast. I’m your host, Spencer Acain. In this series, we talk to experts all across Siemens about a wide range of AI topics and how they’re applied to different technologies. Today I’m joined once more by Richard Barnett, Chief Marketing Officer for Supplyframe, as we continue to our discussion on Supplyframe’s applications of AI. As we continue our discussion from last time, we were talking about how data was a key element of any AI solution. I mean, you really can’t have AI without data, of course. So with that in mind, what are you all leveraging to get the insights Supplyframe creates? As you mentioned previously, you guys were what, six, eight months ahead, predicting the troubles the automotive industry faced with the crash while they were only about three weeks ahead on their predictions. So how were you able to do that? What kind of data are you gathering to be able to get such long-reaching predictions?
Richard Barnett:
Right. I mean, I think there’s different, as you said, there’s a variety of different kinds of data and data signals. Some of it’s derived, some of it has a data life cycle that is changing every minute, every second or is only being updated every few months. And so there’s this interesting both data and volume, but the variety of the data sets that we’re looking at, it has its own interesting challenge to manage, normalize, and then train and then aggregate and then predict. Basically, that’s the pattern. So some of that data is user-oriented behavior. So it is engineers or supply chain professionals. What is their search behavior? What is their popularity of engagement around advertisings or technical content downloads or in community sites that are signaling popularity based on topic or based on end application?
So that’s user engagement, very similar to what we’d be measuring or building algorithms for in general around say social engagement or search methods. So there’s that zone. Then there’s another zone, which is more attribute driven, which is every part component has something like 40 to 50 unique parameters that are associated and vary. The types of parameters vary by the type of component. If it’s a resistor or diode, you’ve got different parameters you’re looking at than if it’s an IC or a chip in terms of power consumption, amperage, whatever it might be. But the idea that you have multiple technical parameters that both give you an opportunity to both normalize and aggregate through the dimension of that attribute like parts, components for applications. But then also you can look at those same attributes in terms of search behavior because there’s a certain set of search criteria that’ll be on the same attribute dimension. So you find these unique ways of taking structural and attribute-based dimensional data with search and user engagement behavior.
And that gives you this way of linking and sometimes discovering new clusters or new similar like patterns that are not obvious before. But we also have that user behavior and company engagement activity by geography, by industry. So that’s important so we have a geographic distribution view and an industry view broadly. But we’re also looking at as somewhat static information as new parts and components are introduced, there’s a life cycle to each of those parts or components. Maybe new companies will announce and build one, two, three major new product introductions and a family of components or products that they’re launching to market every year. And then that gives you a lifecycle view of those products in transition. And each one of those new product introductions may or may not be successful in terms of ramping to volume because there’s so many different components and so similar or directly equivalent components that you need to sort through the life cycle of those parts and components.
And then there’s the market, so supply market dynamics. So you’re looking at the relationship between more supply chain, cost, lead time, inventory. And that’s also very interesting because there’s typically different correlations that you see in a spot market that’s highly constrained. Price is not as sensitive. You’ll see brokers were taking advantage of a lot of the market imbalances and sometimes charging 1,000 times, 5,000 times what the piece part price would’ve been prior to COVID. Because it was a critical part component that companies were willing to spend that much money because it was in critical need. That’s very different from when you have normalized lead time, what the price or cost sensitivity is. Typically the prices and costs are fairly stable. They don’t move a whole lot, maybe 1%, 2%. Maybe there’s a price bell curve and cost curve that we’re seeing across companies that have higher volumes versus companies that are buying one or two.
You’re going to see this distribution of what’s good, better and bad, you know what I mean, across each of those components or light categories. And so when you combine an economic view of price curves and lead time and supply market offsets, and you understand the correlations between them with the technical content and then the actual segmentation basically of engineers and by industry, by geography, by application, generally, you have a really interesting rich data set that you can actually start building new predictive models around and or discovering new relationships that are emerging that you didn’t see before. And that’s pretty fascinating.
Spencer Acain:
I mean, it sounds like you’re leveraging just a hugely wide and rich data sets here from everything from the consumer, not quite consumer level, but the individual engineering level all the way up to the way the companies as a whole are positioning themselves and doing things. So I guess it leads into my next question is do you see this type of AI technology that you’ve got going here, this predictive model and huge data gathering expanding beyond your current industry, the electronic parts industry that Supplyframe is built on right now?
Richard Barnett:
Well, I think it’s a great question. I mean, we are very focused and excited about that. I mean, one of the things that we’re looking at is we’ve primarily been engaging around electronic engineering related hardware, software. We’ve moved slowly into some adjacent industries like MRO and MRO distribution, supporting some customers moving in that direction. But really if you think about the bigger picture, it’s really mechanical design and the broader ecosystems around additive manufacturing and the ecosystem around mechanical and custom design, CNC machine fabrication, the more mature side of some of these industries like an industrial equipment or heavy equipment. There’s this parallel universe of engineering activity and mechanical that then bleeds over into also building and construction. And that engineering design community more broadly is all open to us doing exactly the same thing we did broadly in electronics.
So how do we understand that ecosystem? How do we understand what are standard mechanical parts or patterns of attribute-based design that’s similar but maybe similar manufacturing process, but obviously maybe a different unique design? But very similar from a cost or from a ecosystem of suppliers and manufacturers that they might be working with. And then build out that same more complete picture where you start looking at engineering intent and supply market conditions and trying to build predictive models around where there might be indirect cost drivers. Because this cost volatility, by the way, extends into commodities that are input costs, not just the actual, say, finished electronic part or mechanical part. So understanding those input cost drivers is really interesting. You know what I mean? And then you start overlaying new sets of same pattern but tuning those data models for those unique categories or segments. And now you have a really incredible view of almost 80, 90% of what’s going into almost any discrete manufacturing product in the world across almost every industry. And that’s a huge opportunity because supply market volatility, capacity challenges, responding to these broad supply chain disruptive events.
Spencer Acain:
That sounds fascinating, but are there any other concerns or challenges that you see when extending the supply frame model of AI and insights into other aspects of mechanical design?
Richard Barnett:
With mechanical, the other part of it that’s really interesting is when you start thinking about that ecosystem, those suppliers, the global discrete manufacturing spend is over $4 trillion per year. I mean, and it’s growing healthy. I mean, global manufacturing continues to grow and diversify. But we’re facing new headwinds. The same broad supply chain challenges that are impacting electronics impact everyone else in core manufacturing, including changes in geopolitical trade, tariff policy, diversification of risk to sources of supply, moving to maybe a little bit more of a regional supply chain strategy, China Plus One strategy. I mean, all these disruptive changes all require us to be smarter, faster, more predictive and really think about insights that impact the entire product life cycle from engineering design all the way through the ramp to volume and the global go to market for these products and understand risk and how do you design in resiliency at the point of design and build more flexibility and intelligence to respond to these broad impacts.
To me, it really requires applied AI to extend into these new adjacencies almost and mechanical and these other areas within discrete manufacturing are definitely we’re focused on.
Spencer Acain:
Yeah, I mean, it sounds like you guys have a real roadmap here to try to expand out of just one area and into maybe, I would dare say, design in general, the complete package. And before we wrap this up, anything else interesting you’re working on either now or in the future that you’d like to share?
Richard Barnett:
Yeah, there’s some things I can’t talk about in detail, but we’ve had some interesting quick little spreads to test out things like a ChatGPT driven interface trained on a more discrete model that might change the way that you engage and learn and surface intelligence for all the intelligence that we’re talking about, but in a new way so that we’re reimagining maybe what is the user experience? What is the ways that we communicate and discover new insights that is based on the same data sets and the same AI models, but it’s maybe really fast and easy to consume and understand and scales that access to that intelligence in ways that you’re just not able to do through the current channels that we have to distributed and bring on folks to our portfolio of SaaS solutions or our market intelligence offerings.
So we’re really thinking and imagining the future and trying to stay abreast of, we’re going through another disruptive cycle again where folks are reimagining things they took for granted again, and we want to play there too. And so I think the stay-tuned message is not just ourselves, but I think we’ll see this in the industry as new forms of, think of it as user interface. New ways to access and play with and ask and engage more conversationally what used to be in the domain of very complex, hard to access insights and information that now we’re playing around with as consumers. Well, we really should explore what we can do there in the business community as well. So that’s the last comment I’d make is that I think that’s got a lot of runway and a lot of fun to explore and innovate in that experience as well.
Spencer Acain:
That sounds like an admirable goal to be sure. Bringing all of your knowledge and all of your abilities to everybody. Just bringing it into an accessible format. And I think that’s a great way to end this here. So thank you, Richard, for joining me here.
Richard Barnett:
Thank you, sir. I appreciate it. It’s a great opportunity and look forward to our next conversation.
Spencer Acain:
Absolutely. So once again, I have been your host, Spencer Acain, and thank you for joining me on the AI Spectrum podcast.
Siemens Digital Industries Software helps organizations of all sizes digitally transform using software, hardware and services from the Siemens Xcelerator business platform. Siemens’ software and the comprehensive digital twin enable companies to optimize their design, engineering and manufacturing processes to turn today’s ideas into the sustainable products of the future. From chips to entire systems, from product to process, across all industries. Siemens Digital Industries Software – Accelerating transformation.