The future of simulation with AI
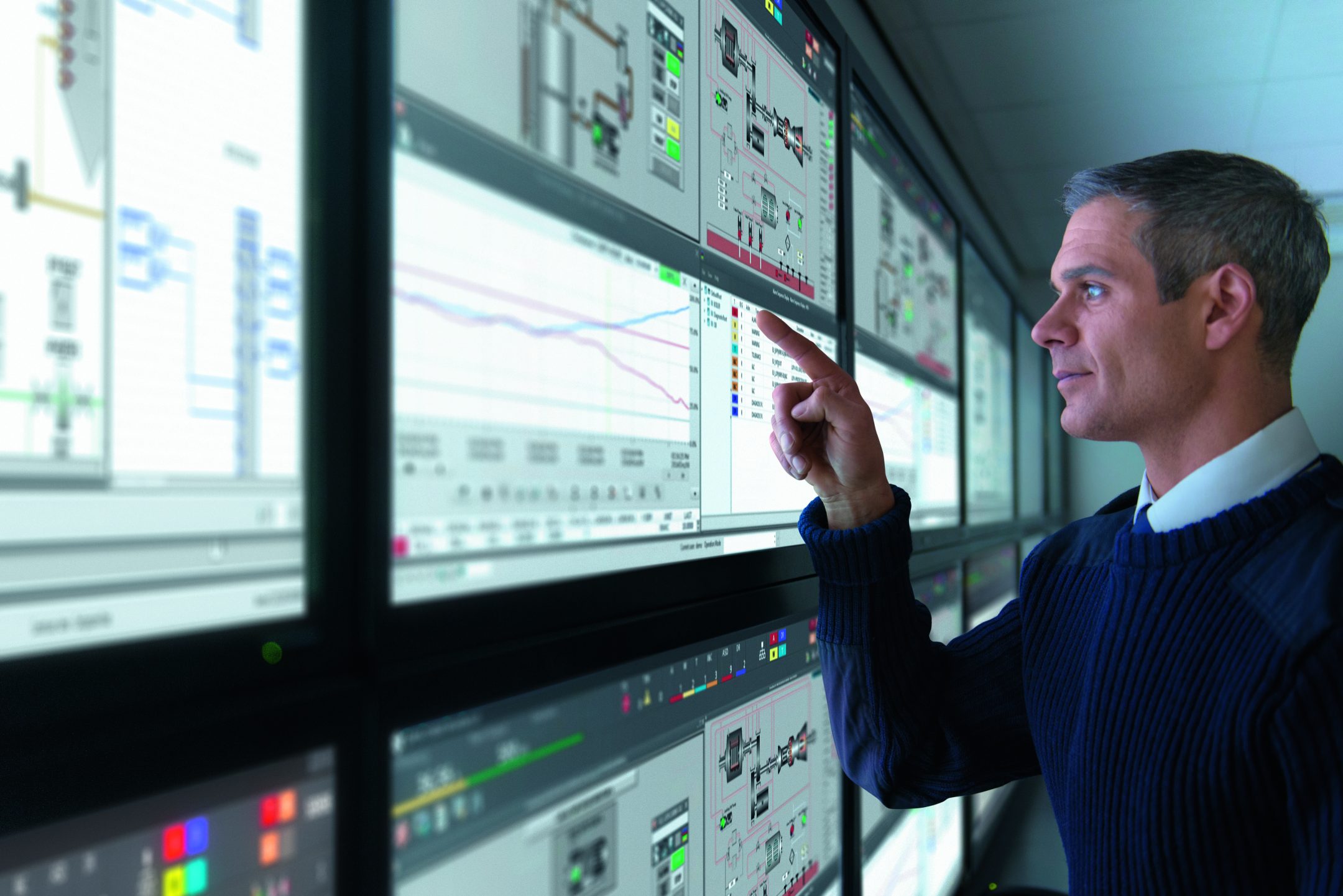
Recently, I continued my conversation with Dr. Justin Hodges, an AI/ML technical specialist and product manager for Simcenter. We focused on the ways AI is driving increased interconnectedness in technology and its potential to change the landscape of physics-based simulation. Looking toward the future, Justin also offered a few remarks on where he sees AI going and the challenges of trying to predict the future of such a new, disruptive technology. I’ve highlighted a few interesting points from our discussion below. The full podcast is available to listen to here or read here.
Looking to the future at what impact AI will have is a daunting task which Justin compares to trying to guess the impact of the iPhone before its release. However, based on his experience, he foresees AI changing the way we handle knowledge, especially when it is distributed across many people and databases. With AI to handle the work of analyzing the vast quantities of data being collected every day, far less useful information will fall through the cracks. Justin gives the example of an engineer wanting to run an expensive test, when instead an AI could collate past data and conclude the test is likely going to be a waste of time, saving both time and money.
To start things off, Justin expanded on the ways AI enables interconnectedness and cross-pollination between different tools and why that’s valuable – an important topic we have discussed previously. Specifically, he examined the need for standardization between different tools, whether the tools are from a single vendor or multiple vendors.
By building around a set of standards, it is possible to lower the bar for entry so even non-AI experts can make use of AI tools. This is an important goal for Justin and his team, since the majority of tools that incorporate AI and ML are targeted at experts in other fields, such as engineering, design, or simulation. By bringing greater accessibility to AI, the more people will be able to use it and that, in turn, will enable AI to continue to develop from the inflow of new ideas and knowledge.
Our conversation then shifted to the topic of AI in physics-based simulation, such as physics informed neural networks or PINNs. When it comes to using AI for something like inferring physics, there are some substantial challenges that must still be overcome. As in many fields, data is a big factor when it comes to PINNs. Right now, simulations can provide data for model training and, by treating that data as 100% accurate, the AI has a wealth of information to train with. However, it may still be desirable to add other, real sources of data into the training process as well.
Additionally, the viability of AI as a solution in this space depends heavily on the problem it’s addressing, with better results being generated when the AI is trained to work on a smaller more focused problem set. This tradeoff between creating a more general model vs. creating a model that is highly accurate means that PINNs are simply not cost competitive with existing techniques due to their vast data demands. Training the model to the required accuracy may actually be more costly than simply running the simulations normally. However, there is still potential for substantial benefit. For instance, an AI model, once trained, could offer near instant results compared to the long run times inherent to physics-based simulation and models could be continuously reused and refined, reducing the cost of usage in later projects.
Artificial intelligence offers a host of potential benefits which can be hard to even conceive of at times. Everything from simple time savings to full-featured design assistants and more are possible as AI continues to make deeper inroads in different fields. PINNs too, represent the potential for a dramatic shift in the way physics-based simulation is handled, opening the door to integrate high resolution simulation into tools that would traditionally make do with low fidelity approximations. All these innovative techniques are just the tip of the AI-iceberg and only in the years to come will the full impact of what AI is capable of be felt.
Siemens Digital Industries Software helps organizations of all sizes digitally transform using software, hardware and services from the Siemens Xcelerator business platform. Siemens’ software and the comprehensive digital twin enable companies to optimize their design, engineering and manufacturing processes to turn today’s ideas into the sustainable products of the future. From chips to entire systems, from product to process, across all industries. Siemens Digital Industries Software – Accelerating transformation.