The other AM of additive manufacturing
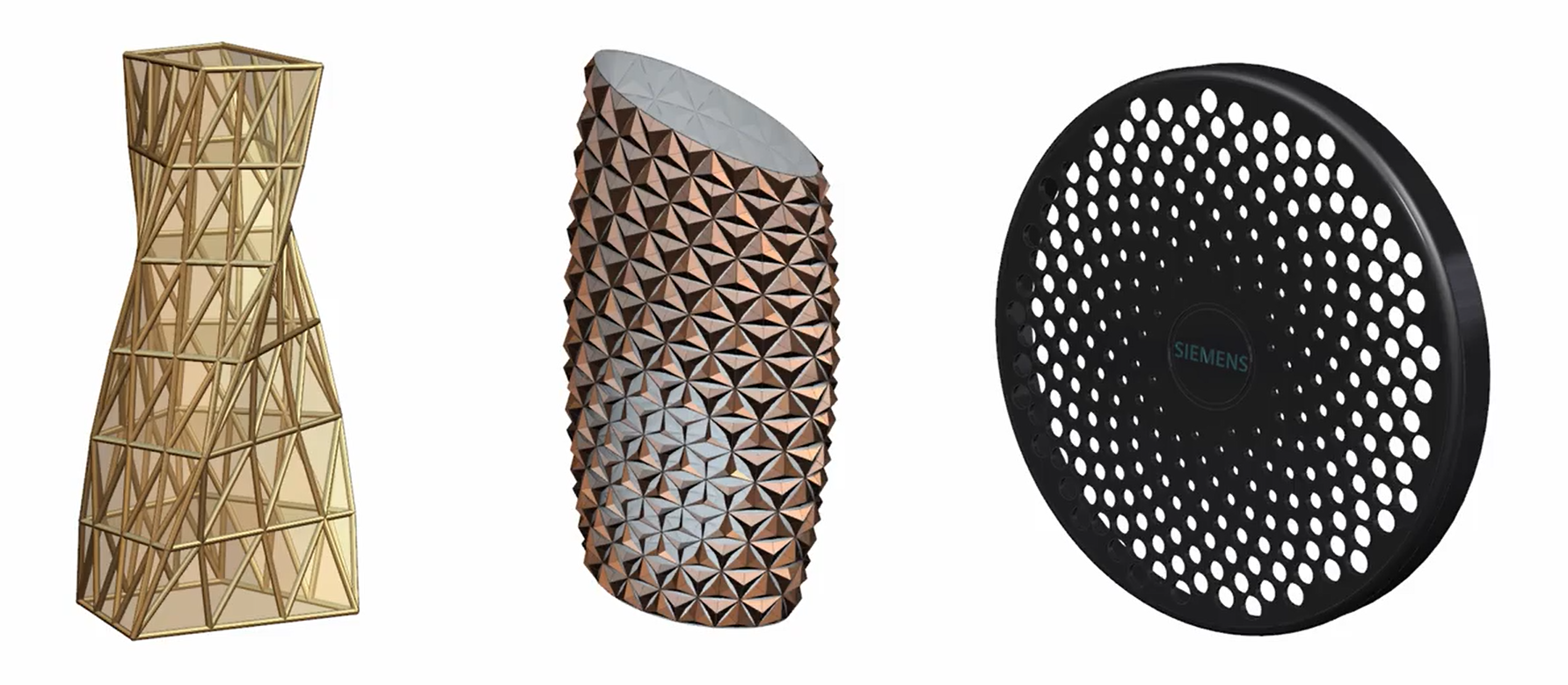
Creating better geometric features are essentially what every mechanical designer is working towards. Better can be defined a number of different ways, through performance, manufacturing cost, design simplicity, or any number of additional metrics. But, as the geometric designs are improved and a greater number of competing metrics are used to define better, the process to create that design becomes more complex. And this is especially true for any applications involving additive manufacturing (AM), it provides a wider solution space than previous manufacturing methods and more variables to the development process. Overcoming this increased complexity and variability is oft-touted as solved with topology optimization solutions and artificial intelligence-aided design space exploration. And that is a great engineering path for some applications, but it takes some of the engineering work away from the engineer, unlike another major path – algorithmic modeling.
What is algorithmic modeling?
The complexity of today’s geometric models is growing too quickly for designers to keep pace using traditional modeling tools. Spending more time and resources doing a direct modeling application, algorithmic modeling acts as a translation between the designer and the geometry to create repetitive and definable features in a few clicks. The geometric capabilities afforded by algorithmic modeling are not exclusive, in fact users have been defining geometry based off of complex mathematical equations and manual code for years. Instead, the value comes from the ease of use and deployment – a surface pattern can be applied to different objects without the need to recode the object geometry and any changes that do need to be made can be completed with a graphical interface of operations and variables. Algorithmic modeling empowers users to harness the value in complexity by augmenting a human’s design abilities rather than replacing them.
Algorithmic modeling in AM
This capability is not entirely different from topology optimization, however. They both benefit AM in creating more performant components, but they are not limited to AM aside from the most innovative applications. External features can often be created using more traditional manufacturing methods, but internal geometries are where AM reigns supreme in complex components. A great example for algorithmic modeling is in creating lattice structures inside of a part, some tools can automatically fill a body with specific infill patterns, but applying algorithmic modeling makes the process more easily customizable. A lattice might be chosen for printability or structural rigidity, but it can also be optimized with how frequently the pattern repeats, the thickness of the outer shell, or even the minimum resolution possible with your manufacturing machine.
It is one tool in the toolbox
Value gained with algorithmic modeling, whether it is used with AM or not, is great for complex geometries but it is one tool in a greater solution. It can be combined with design for additive manufacturing as discussed briefly, but it can also be augmented with design space exploration activities as seen with topology optimization. Since all of the rules in the algorithm are parameterized, an iterative design-simulate-verify loop can be implemented to find optimal geometries for a task. When creating a lattice structure for a heat exchanger, you may want to find the fight balance between surface friction, material cost, and thermal transfer performance. The system could try a variety of slightly different designs to zero in on the right mix for the component requirements, just as topology optimizations do for fluid flow and weight studies.
Creating an open platform
These are just a few of the possible applications of algorithmic modeling, however, and it will flourish even more once it is readily available to a diverse group of users and partners. An upcoming release of NX will enable extending the algorithmic framework with custom features, this will enable variables and parameters that are either not directly supported in NX or wholly custom ones specific to your business or application. And commonly used custom functions can be condensed into simple in-out nodes within the algorithmic modeler as Snippets in NX. Just as before these constructed algorithms are valuable because of their reusability, if you create a workflow with variables those can be changed for a new component in a few clicks and allowing more time for optimization and test rather than tedious modeling of something previously designed.
A future of possibilities
There was an amazing presentation from Taylor Anderson from NX on this topic at Realize Live this year, focusing on the role of a technology partner – ELISE. But if you couldn’t make it this year, make sure to watch out for the next release of NX coming out this Summer. I’m excited to see what the next step in algorithmic modeling may be, even if it is not a part of an additive manufacturing solution.
Siemens Digital Industries Software is driving transformation to enable a digital enterprise where engineering, manufacturing and electronics design meet tomorrow. Xcelerator, the comprehensive and integrated portfolio of software and services from Siemens Digital Industries Software, helps companies of all sizes create and leverage a comprehensive digital twin that provides organizations with new insights, opportunities and levels of automation to drive innovation.
For more information on Siemens Digital Industries Software products and services, visit siemens.com/software or follow us on LinkedIn, Twitter, Facebook and Instagram.
Siemens Digital Industries Software – Where today meets tomorrow