Detection and mitigation of AI bias in industrial applications – Part 1: Background and definitions
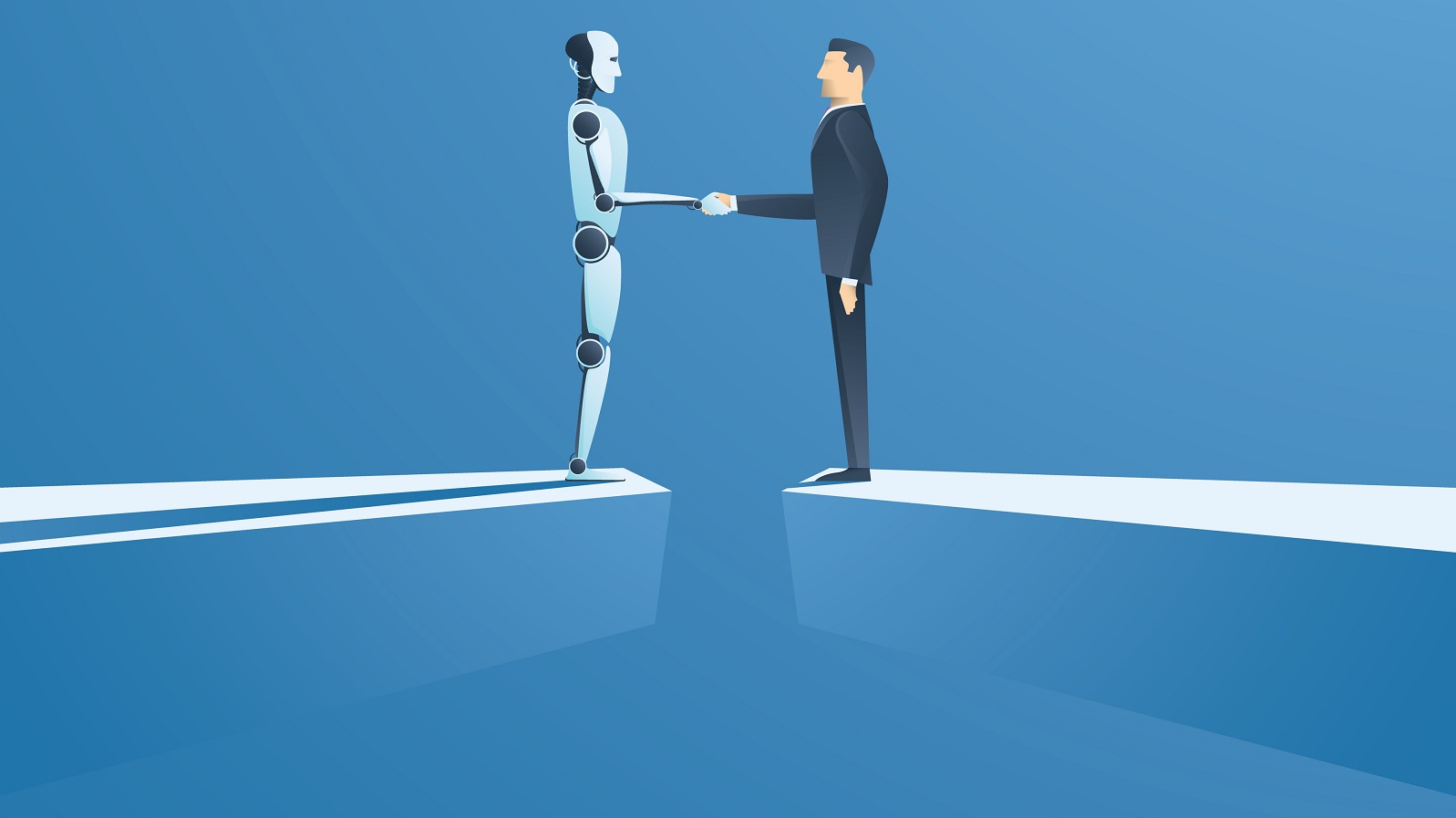
Traditional machine learning techniques optimize for accuracy only, not fairness or avoiding unexpected/harmful bias. Current advances in AI are both extraordinary and theoretical, with fairness and bias issues (along with lack of transparency, security, and privacy) limiting practical applications.
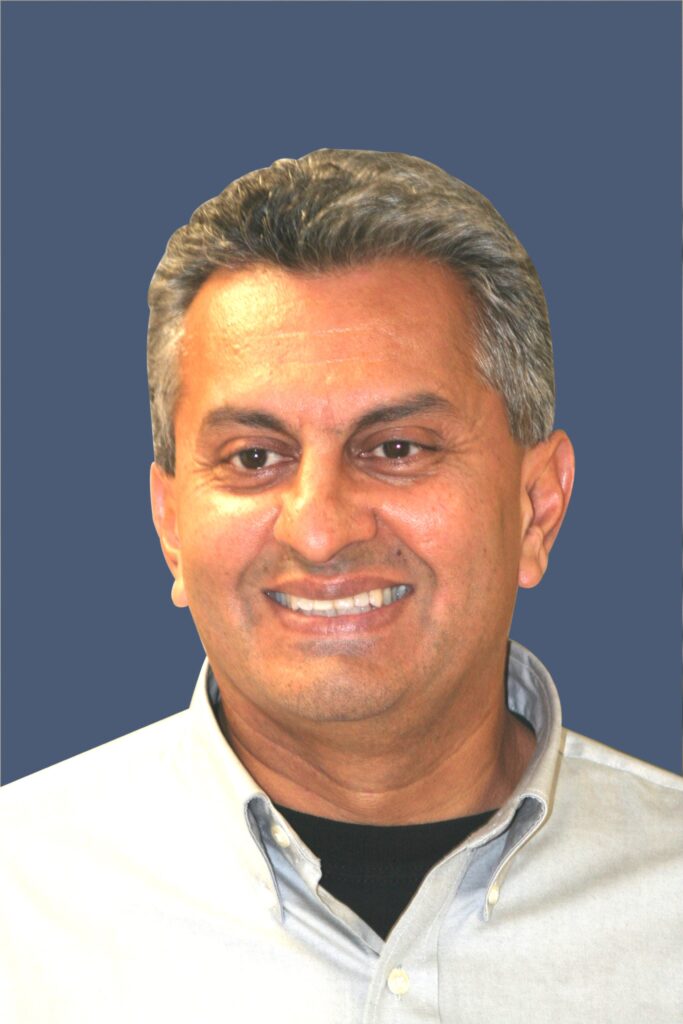
In this first part of a three-part series, I am discussing bias in the context of trustworthy Artificial Intelligence (or more specifically, Machine Learning) models[1] [2]. At Siemens, bias detection and mitigation techniques are highly relevant to our organization’s use cases seeking to automate decision-making in products and/or processes. For instance, AI-based recommender systems we are currently building for industrial applications could easily suffer from harmful bias if we don’t take what is stated here seriously.
Note that trustworthy AI models contain many “desirable” biases because bias (in its broadest sense) is the backbone of machine learning. A part recommender model will correctly predict that components with a history of breakdowns are biased towards a rejection result. The International Organization for Standardization (ISO) defines bias in statistical terms: The degree to which a reference value deviates from the truth [3]. This deviation from the truth can be either positive or negative, it can contribute to a harmful or discriminatory outcome, or it can be beneficial towards a future we want if properly managed. We should direct our AI models away from any harmful bias and, if appropriate for the use case, towards a desirable bias.
Impact of harmful industrial bias
Decisions have real world consequences. Therefore, whenever an AI model is used in an industrial setting, its correct operation is essential. It is unlikely that technology with “zero risk” can be developed, but managing and reducing the impacts of harmful biases in industrial AI is necessary for developing trust. AI-based systems meant for decision-making or predictive scenarios should demonstrate validity and reliability under specific settings for deployment. Currently, organizations that design and develop AI technology use the AI lifecycle to keep track of their processes and ensure delivery of high-performing functional tools, but not necessarily to identify harms or mitigating them. The goal here is to change the trend by identifying potential sources of bias in industrial applications and introduce mitigation strategies to avoid them at various stages of the AI lifecycle.
To accurately make predictions, machine learning algorithms look for patterns in the training data that are correlated with a particular prediction. If that training data is biased, then the AI model is also biased because it has been trained to fit the biased data. For example, in industrial applications of AI, an algorithm might discover the pattern that a particular version of a part seems to be correlated with increased breakdown of a machine. This can be the wrong conclusion, however, if its predictions are based solely on data taken at a specific time of the year and/or from a small sample of machine operators. If training data is collected primarily from a particular category of candidates, or if the training data is annotated and analyzed by a similarly biased group of experts, the artificial intelligence applications based on that model are very likely to contain harmful inadvertent/unconscious biases.
AI transparency and accountability
As companies look to bring artificial intelligence into the core of their business, calls for greater transparency and accountability of AI algorithms are rising [4]. The problem set surrounding bias detection and correction in AI systems is complex. NIST identifies nearly 40 sources of bias [5], which must be consolidated and defined in the context of model generation and implementation. Figure 1, an adaptation from [6], is a first attempt at doing just that for industrial applications.
In future blogs, we will continue to explore the detection and mitigation of AI bias, focusing on key points of bias for industrial applications as shown in Figure 1. We will also review several real-world industrial examples to provide clear context for the discussions.
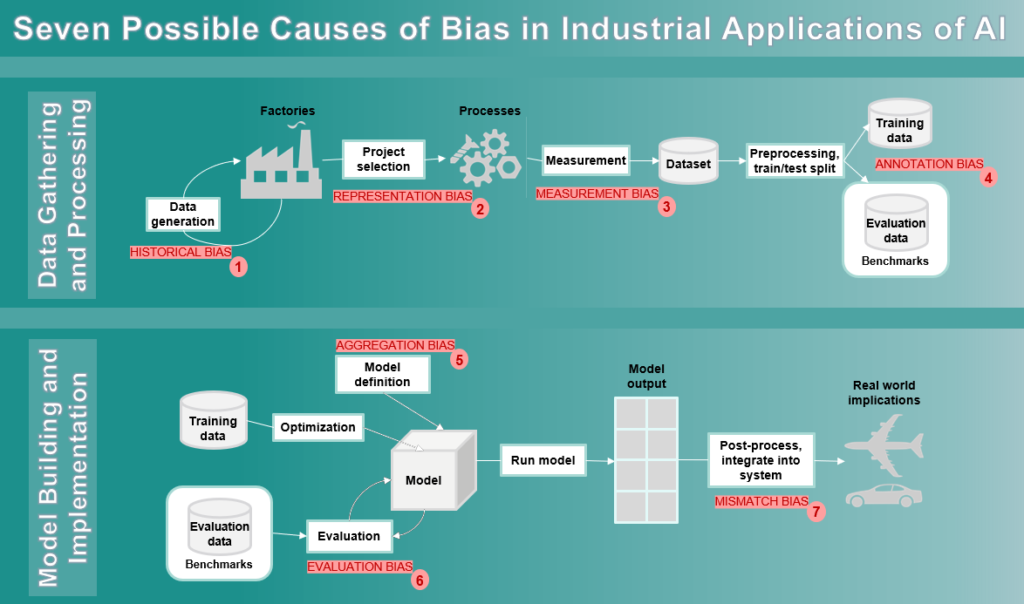
Software solutions
Siemens Digital Industries Software is driving transformation to enable a digital enterprise where engineering, manufacturing and electronics design meet tomorrow. Xcelerator, the comprehensive and integrated portfolio of software and services from Siemens Digital Industries Software, helps companies of all sizes create and leverage a comprehensive digital twin that provides organizations with new insights, opportunities and levels of automation to drive innovation.
For more information on Siemens Digital Industries Software products and services, visit siemens.com/software or follow us on LinkedIn, Twitter, Facebook and Instagram.
Siemens Digital Industries Software – Where today meets tomorrow.
References or related links:
- Mohsen Rezayat: Trust, the basis of everything in AI, November 2020.
- Harvard Business Review: We need AI That is Explainable, Auditable, and Transparent, October 2019.
- ISO, Statistics — Vocabulary and symbols — Part 1: General statistical terms and terms used in probability, 2006.
- Mohsen Rezayat and Ron Bodkin: The Future of AI and Machine Learning, October 2020.
- NIST special publication 1270: A Proposal for Identifying and Managing Bias in Artificial Intelligence, June 2021.
- Harini Suresh and John Guttag: A Framework for Understanding Sources of Harm Throughout the Machine Learning Life Cycle, December 2021.
About the author: Mohsen Rezayat is the Chief Solutions Architect at SIEMENS Digital Industries Software (SDIS) and an Adjunct Full Professor at the University of Cincinnati. Dr. Rezayat has 37 years of industrial experience at Siemens, with over 80 technical publications and a large number of patents. His current research interests include “trusted” digital companions, impact of AI and wearable AR/VR devices on productivity, solutions for sustainable growth in developing countries, and global green engineering.