Why today’s engineering challenges are difficult to overcome
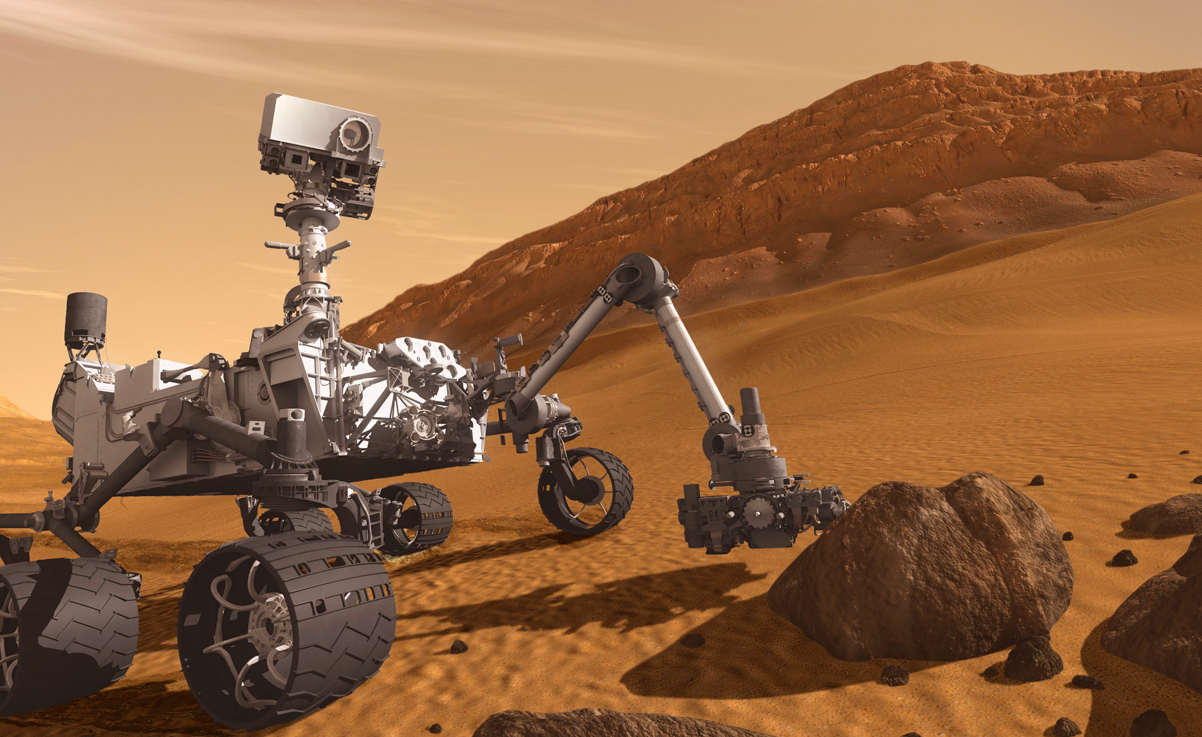
Imagine you’re designing a product that will literally be out of this world. It’s going into space: to Mars, to be exact. It’s an expensive and ambitious feat. You have to ensure the product works perfectly when it’s 225 million kilometers (140 million miles) away.
The Curiosity rover team faced this dilemma and a host of engineering challenges. How could they design something they couldn’t physically test in its actual operating environment until it was millions of kilometers away?
The team needed to simulate the landing thousands of times and be confident everything would work. These simulations needed to account for hundreds of factors that would affect the landing, including the difference in gravity, the atmosphere, the red dust and the temperature changes throughout Martian days and seasons.
They also had to use systems thinking to ensure different systems could properly function and evolve. For example, the Curiosity rover had to be able to receive a signal, navigate to a rock, have an arm drill into the rock, retrieve samples, move the samples to a chemistry analyzer, perform an analysis and then send that data back to Earth. One failing part could threaten that task, or even the entire mission.
The simulations also needed to account for how the rover would act after being on Mars for a while. But there were no mountains of usage data to leverage for informing future variables in simulations.
Few, if any, teams had ever dealt with the extensive engineering challenges the Curiosity rover team had.The team needed something that would be capable of handling all of these factors and to have those factors evolve. They needed something that included the knowledge of how long the rover had been on Mars and the distance it had traveled. It was essential to have something that would include that degraded performance information and be able to update that information as data is received.
This was a massive engineering problem, but the team was successful. The Curiosity rover landed on Mars in 2012 and has been plugging away since then. The rover even has its own Twitter account with regular updates about what it sees and what it’s doing.
The Curiosity rover team relied on Siemen PLM’s product portfolio to overcome the engineering challenges of designing a product for Mars.
CAE was crucial to the Curiosity rover’s design and engineering process.
Engineering challenges in today’s manufacturing realm
Like the Curiosity rover team, manufacturers are facing many new engineering challenges. They’re working with more complex systems and “unknowns” they’ve never worked with before. They’re designing autonomous cars that could one day talk to each other and to traffic control systems; they’re designing aircraft made of lightweight composites.
These new processes and unknowns are giving manufacturers additional hurdles to navigate as they rush to get their innovations to market. They also must prepare for greater access to usage data in the new world of the Internet of Things. How can they use this data to get a competitive edge?
Product development used to be simple. You created a draft of the product, and then you built and broke it until you got it right.
Today’s mindset is to design and then verify that the design will behave. Manufacturers use 3D representations to visualize assemblies and dive deep into parts to verify specific performance attributes. They validate with CAE and tests.
This process sounds fine, but it’s incredibly problematic: everyone is designing, building and analyzing product models in their own groups.
As smart products hit the market, the process doesn’t stop after you deliver a product: every customer provides feedback and data from the field. And as these products saturate the market, this “siloed” process won’t cut it.
In fact, it dooms to you to failure. It doesn’t allow you to incorporate data from the field. You don’t get a clear, holistic view of what’s happening across different sources.
To overcome these engineering challenges, manufacturers need something richer than 3D representations. They need what we call a digital twin, a digital representation that can predict all performances of the product, as a whole system, throughout each step of the development process. It can also evolve over the product’s life. By staying in sync with the product as a physical entity, the digital twin helps manufacturers answer questions about how the product behaves in the real world.
We want to shift this process so manufacturers have the data and models that help them predict what next generations need to be as they design, not as they verify. This change gives manufacturers the ability to represent an actual reality, not an ideal reality. By doing so, they get the answers they need faster and they have more confidence in those answers – a must in a highly competitive market.
Manufacturers will have a process that represents the product as a system, including its electronics, functional behavior, logic and software. Test data, benchmark data, simulation results, prototype test data and usage data will flow in. Every previous simulation and its results will be incorporated into the next simulation. And, the process will have a predictive analytics layer to help manufacturers confidently calculate what happens next.
Predictive engineering analytics to conquer engineering challenges
This is the future we see, and manufacturers will thrive in this future by implementing predictive engineering analytics. It’s the application of multi-discipline simulation, testing, intelligent reporting and data analytics to develop digital twins that can predict behavior across all performance attributes during the product’s lifecycle. It includes tactics and tools that help manufacturers evolve their traditional design verification and validation processes into a more predictive one. Predictive engineering analytics, or PEA, is core to Systems-Driven Product Development.
Ultimately, this kind of predictive engineering analytics will help manufacturers deliver innovations faster and more confidently.
Siemens PLM recognizes the need to update this process, and its recent acquisitions of LMS and CD-adapco are central in the company’s predictive engineering analytics and digitalization vision. Through internal and external investments, Siemens has built vast expertise in 1D and 3D simulations, software and hardware testing and advanced capabilities with computational fluid dynamics and multi-discipline exploration. This expertise allows us to offer customers the tools they need so PEA can help them have a successful business.
Tell us: What are some of the most frustrating engineering challenges you face?
About the author
Dr. Jan Leuridan is the senior vice president of simulation and test solutions for Siemens PLM Software, a business unit of the Siemens Digital Factory Division. He also serves as CEO for Siemens Industry Software NV. In the early 1980s, Dr. Leuridan joined LMS International in the position of Chief Technical Officer for the company, and member of the company’s board of directors. Following Siemens’ acquisition of LMS in 2013, he assumed his current role as leader of the simulation and test solutions business segment within Siemens PLM Software. He has an engineering degree from the Department of Mechanical Engineering at the University of Leuven in 1980. He also has a M.S. (1981) and a Ph.D. (1984) from the department of mechanical and industrial engineering at the University of Cincinnati. He lives in Leuven, Belgium.