Inside The Forecast Factory: how AI is set to revolutionise weather forecasting
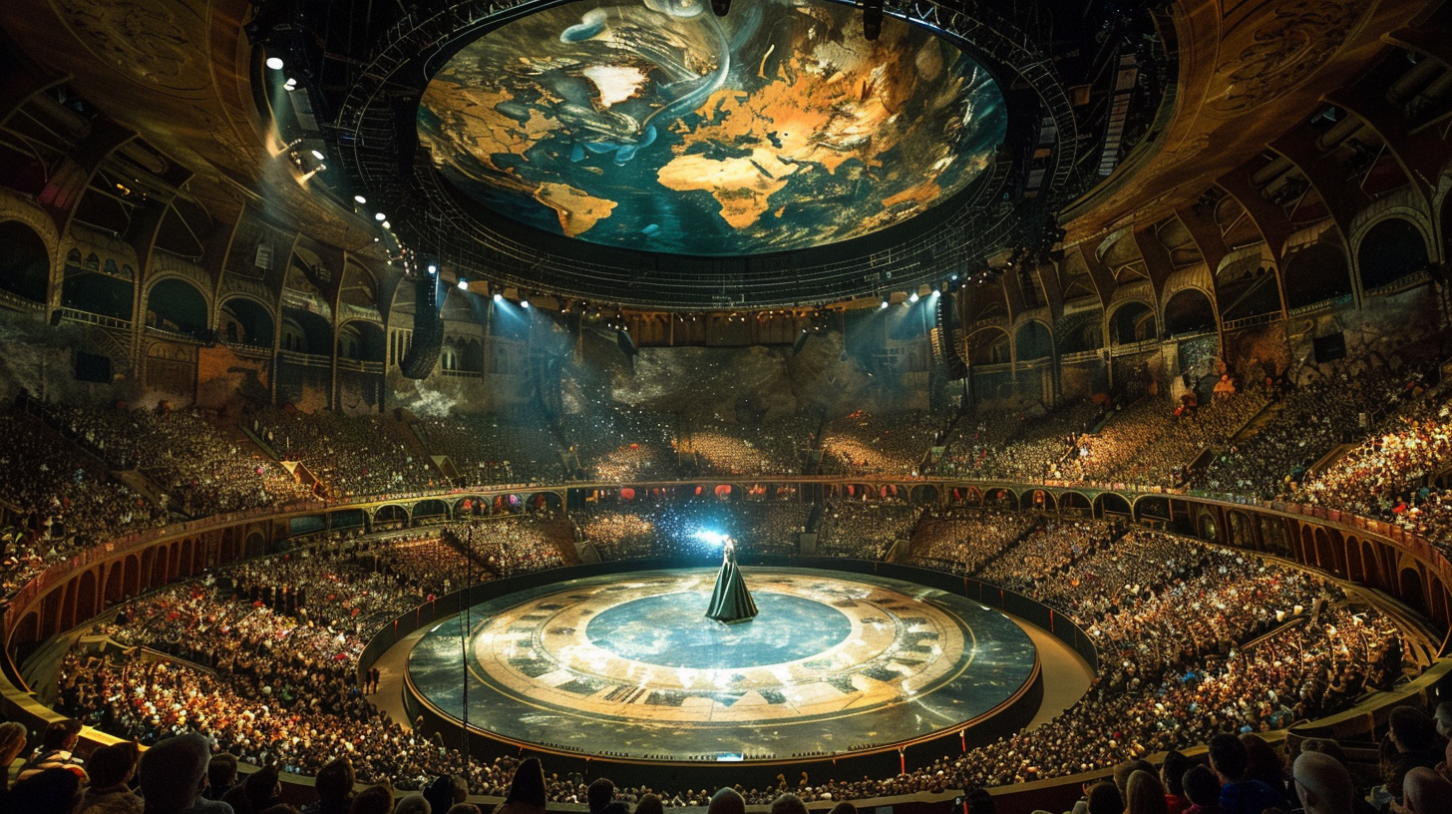
For most of the past 108 years we have been using the most powerful computers available to forecast the weather (although for the first three decades “the most powerful computer” was actually a human).
Most of us take the availability of relatively accurate localised weather forecasts for granted, using them to make decisions in our everyday lives. “Should I cancel the garden party next weekend?”; “Will I get sunburned during my marathon?”; or “Shall I take an umbrella to work?”. More importantly it allows people to answer questions like “Will my home be flooded?”; or “Will the tornado hit my town?”
Frustratingly, although weather forecasts provide a degree of certainty, they never tell us exactly what the weather will be like in the future, usually delivering stochastic predictions such as “there is a 40% chance of rain” or “wind speeds will be between 16 and 20 miles an hour”.
This shouldn’t be too surprising. Weather forecasting is, at its heart, an attempt to apply order to chaos. It’s the meteorological equivalent of trying to herd cats, if the cats were made of water vapour and easily distracted by the flapping wings of a butterfly in Brazil.
In this blog we’ll explore how those forecasts are made today, and how AI might improve weather forecasting in the near future.
In the beginning
Weather forecasting has its roots in ancient civilizations, where the observation of astronomical and meteorological events guided the understanding of seasonal weather changes. By 650 B.C., the Babylonians were attempting to forecast short-term weather by examining cloud formations and optical phenomena like haloes. Around 300 B.C., Chinese astronomers had crafted a calendar that marked the year with 24 festivals, each linked to specific weather conditions. By 340 B.C., the Greek philosopher Aristotle contributed to this evolving field with his work, Meteorologica, which explored the nature of rain, clouds, hail, wind, and more, while also touching on astronomy, geography, and chemistry.
Although these methods were sometimes correct – they weren’t often reliable.
That changed in 1904 when Norwegian academic Vilhelm Bjerknes derived the primitive equations of meteorology and proposed a numerical method to solve them. In 1917, whilst working in an Ambulance Unit on the Western Front of the First World War, Englishman Lewis Fry Richardson attempted to solve those equations to provide the first numerical weather forecast.
It was technically a “hindcast” rather than a “forecast” because Richardson used weather observations recorded by Bjerknes at 7am on May 20th 1910, to calculate the weather 6 hours later at 1pm. In a pre-computer age it took six weeks to provide six hours of forecast by hand. And then even the results were poor. Richardson’s simulation predicted a rise in barometric pressure when the observed pressure was essentially constant. However, contemporary analysis has demonstrated that the forecast was only wrong because Richardson failed to use smoothing techniques to prevent unphysical surges in the predicted pressure field.
The first CFD, used by Lewis Fry Richardson to predict the weather over Northern Germany over a 6 hour period on May 20, 1910.
Given that Bjerknes’ primitive equations are simply a statement of the Navier Stokes equations, but with the vertical momentum equation replaced by the hydrostatic approximation, Richardson remarkably managed to perform the world’s first computational fluid dynamics simulation 28 years before the first general purpose computer was commissioned.
In his 1922 book “Weather Prediction by Numerical Processes” Richardson imagined a Forecast Factory in which 64,000 human “computers” gathered in a large dome shaped auditorium, in sections representing various regions of the globe. Coordinated by a torch wielding conductor on a central podium these “computers” would provide daily weather forecasts for the whole world.
Lewis Fry Richardson imagined a “Forecast Factory” in which 64,000 human “computers” forecast the weather for the whole world
In 1950 – three years before his death – Richardson was able to witness his dream of a Forecast Factory come true using digital rather than human computers, when ENIAC – the world’s first computer – performed a 12 hour weather forecast using Bjerknes and Richardson’s technique – in 12 hours.
Weather Forecasting Today
Numerical weather forecasting works by taking a set of initial conditions, the weather observed by monitoring stations and satellites, and advancing in small time increments by solving a set of differential equations using an enormous amount of supercomputer number crunching.
Since the 1980s, speed and availability of computer processing has meant that forecasters are able to use progressively smaller time steps, and finer computational grids. There have also been huge improvements in the quality of measurements used to provide the initial condition of the forecasts, with the proliferation of weather satellites.
Take the example of the UK, which is a small set of rather wet islands just off the North West coast of mainland Europe. The UK’s Met Office uses almost half-a-million supercomputer cores to predict the UK weather. Those calculations are predicated on (and validated by) over a billion points of measured data. All that computational effort – 14,000 trillion operations per second – is to produce forecasts that mostly say “it’s probably going to rain tomorrow”.
In the history of our species no pursuit has gobbled up more CPU cycles than meteorology.
Since the 1990s weather forecasts have used “ensemble averaging” to account for the chaotic nature of meteorology – in that small variations in initial conditions or model parameters can lead to a large change in the weather predicted days later. To account for this, forecasters deliberately randomly perturbed initial conditions to represent measurement errors) across a large number of simulations. A 40% chance of rain means that 40% of simulations predict rain at your location.
This has improved the accuracy, locality and range of forecasts to such an extent that 10 day forecasts today are almost as accurate as 7 day forecasts were in 1981.
In his excellent book The Signal and the Noise: The Art and Science of Prediction (Why So Many Predictions Fail – But Some Don’t), Nate Silver takes a rational look at prediction in all its forms, from earthquake prediction to climate change, from sports betting to the catastrophic failure in the prediction that resulted in the subprime collapse that plunged the whole world into recession. Although he doesn’t examine CFD or engineering simulation directly, he does explore the world of weather forecasting and comes to the conclusion that it is one of the most accurate predictive methods available, mainly because weather forecasters have the opportunity to compare the actual weather against the previous day’s forecast on a daily basis.
Silver also notes that meteorologists do not rely on numerical simulation alone, and use their expertise to manually adjust the results of numerical weather simulations.
The Forecast Factory of the Future
Since Richardson’s first calculation, weather forecasters advance their predictions step-by-step by numerically solving the partial differential equations using lots of number crunching. Despite advances in technology – and obviously refinements in the methodology – the basic technique remains very similar.
Recent developments in deep learning AI are paving the way for faster and more accurate predictions that also consume fewer computing resources. Google DeepMind’s GraphCast is a prime example of this, employing graph neural networks for advanced weather forecasting. Trained on nearly four decades of weather data from the European Centre for Medium-Range Weather Forecasts, GraphCast leverages historical patterns and machine learning to deliver precise forecasts.
Graph neural networks organise data into “graphs” of interconnected nodes that represent atmospheric conditions like temperature, humidity, and pressure at various global locations and altitudes. This network aims to predict the interaction between these data points and their neighbours, charting the future course of weather conditions efficiently.
These approaches still require an epic amount of number crunching, and computation up front to train the algorithm, but are much faster and computationally-less-expensive to actually deploy.
Although AI powered forecasts are still subject to the same sensitivity to input data as physics based forecasts, the ability to perform more forecasts will reduce the amount of uncertainty in the “40% chance of rain type forecasts”.
A weather forecast from Google’s DeepMind GraphCast _______________________________________________________
Strangely, the AI driven Forecast Factory of the future has more in common with the more intuitive style of forecasting – spotting patterns in the weather – pioneered by Aristotle and the ancient Babylonians, than with the purely physics based forecasts inspired by Bjerknes’ and Richardson.
In the grand scheme of things, whether it’s physics, AI or human ingenuity at the helm, the quest to predict the weather is more than just about getting it right—it’s about the thrill of the chase, the pursuit of knowledge, and the endless fascination with the world above and beyond. Here’s to the Forecast Factory of the future—may it be as wondrous and wildly unpredictable as the weather it seeks to forecast.
Now if you’ll excuse me, I need to get the washing in from the garden, before it gets wet from an unexpected rainstorm.