How can we build trust in pre-silicon development for AI in automotive?

The PAVE360 digital twin team has a long track record in working with automotive industry leaders to enable pre-silicon development. An example being our announcement with Arm, where PAVE360 has enabled ecosystem players to develop their SW on the new Arm A270AE, pre-HW.
It takes years to implement new technologies in automotive
It’s well known nothing changes quickly in automotive, as a safety critical industry, a high degree of confidence is required for any data used to test automotive systems. The lack of credible performance prediction, and energy efficiency data, pre-silicon means a wait on hardware and a 3+ year development cycle, much slower than other industries. This is one of the main hurdles in introducing new technology such as AI.
Customers are just starting to adopt digital twin for use cases that include pre-silicon SW development, and pre-silicon CPU performance benchmarking, But, for emerging technology, such as AI based systems, which are required for complex autonomous vehicle functions, a new approach is required.
Proving the performance of AI in automotive cannot be done in isolation
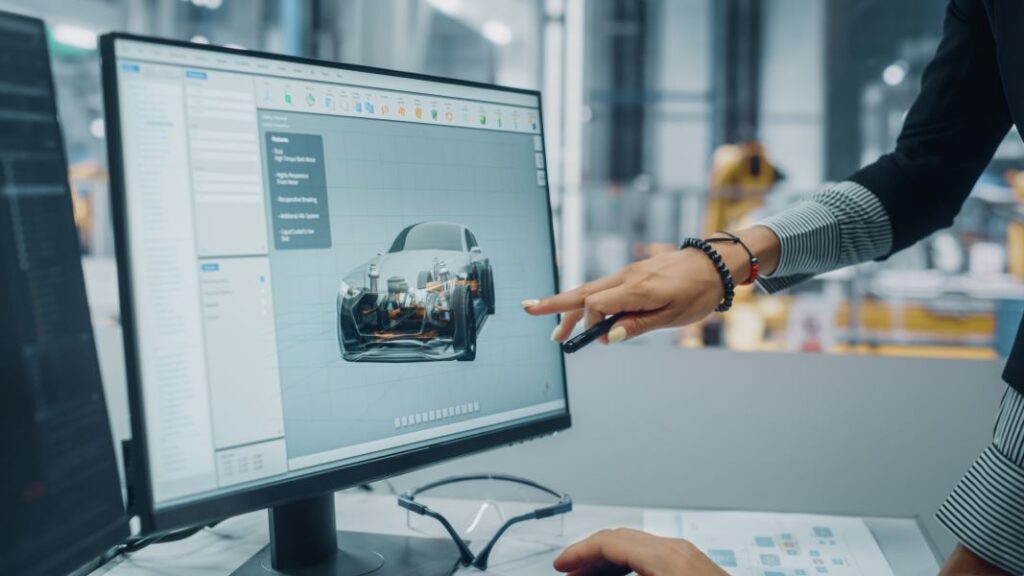
That’s where industry collaboration becomes essential. Take ML Commons, who are a world leader in building benchmarks for AI. They have built an open engineering consortium to improve AI performance in automotive via higher quality data and benchmarks. The MLCommons, MLPerf Automotive benchmark taskforce has taken on the challenge of creating a set of common reference points that will enable OEMs to evaluate the impact of new AI based systems on their computing demands.
This taskforce has just delivered on a major milestone towards building a full automotive benchmark suite, with the release of the new automotive benchmark proof-of-concept.
By collaborating with industry leaders, Siemens can make a difference
Siemens EDA has played their part in the taskforce by lending our PAVE360 pre-silicon development experience, garnered from years of experience in the automotive market, to the benchmark project. By working together with leading industry partners such as Arm, Bosch, Cognata, Knowledge, Marvell, NVIDIA, Qualcomm Technologies, Inc., Red Hat, Sacramento State University, Samsung, Tenstorrent, and UC Davis. We can build confidence in these AI performance measurements for meaningful results, pre-tape-out.
The taskforce is looking for new members to provide their expertise in automotive AI with the goal of having a comprehensive benchmark suite for autonomous vehicles later this year. Why not learn more about the proof of concept so far?