Justin Hodges
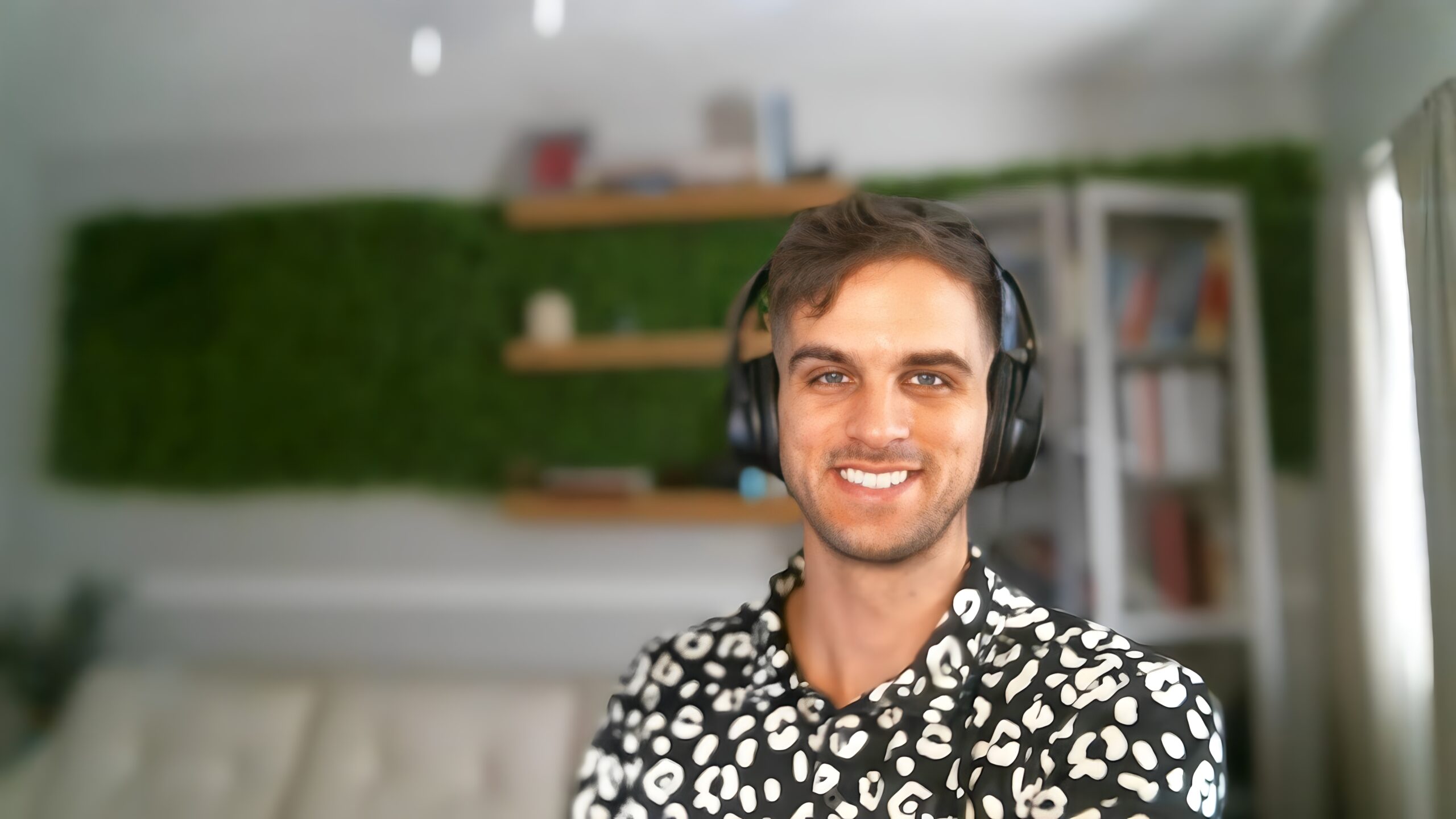
Justin Hodges’ passion for Artificial Intelligence (AI) was ignited in 2017 when he began an internship at Siemens Healthineers. His main task was using computational fluid dynamics (CFD) to model airway diseases within the lungs. Experiments to fuse AI with CFD tools and workflows produced astonishing results and wound up being the central focus of his graduate studies and his eventual AI-focused career at Siemens. Online, he might be best known for the fluid dynamics videos (simulations and otherwise) that he posts on LinkedIn and for his appearances as an AI expert on various podcasts. Yet there is more to Justin than offering hot takes on the hottest trend in technology. Beyond algorithms and datasets, he’s also keenly interested in the simple pleasure of a cup of espresso, perfectly brewed from beans he roasts himself. Indeed, he sees artistry in both coffee and machine learning in CFD, the latter of which is the topic of his newly published book, “Approaching machine learning problems in computational fluid dynamics and computer aided engineering applications: A Monograph for Beginners.” Thanks to Justin for taking a break and talking about his career trajectory, the importance of emotional intelligence in tech-heavy roles, avoiding burnout, and more.
Can you tell me a little bit about yourself?
Throughout my academic journey at the University of Central Florida, spanning my bachelor’s, master’s, and Ph.D. degrees, I was immersed in a lab affiliated with Siemens Energy. This lab had a primary focus on Industrial Turbomachinery, exploring various areas such as fluid mechanics, aerodynamics, turbulence, and heat transfer. During this period, I had the opportunity to participate in an internship with Siemens (formerly CD-adapco). In this role, I worked extensively with Simcenter STAR-CCM+ software, specializing in Computational Fluid Dynamics (CFD) engineering simulations.
Towards the end of my internship, I established a connection with the life sciences director, discussing the prospect of continuing collaborative work beyond the internship. Upon my return to the university, we engaged in CFD applications related to the study and treatment of aneurysms, resulting in published work. I applied for a position within the Siemens Healthineers group in Princeton, drawn by their stellar reputation in the field and from the encouragement of the Life Sciences Director. My biomedical experience played a pivotal role in securing my role in 2017.
Upon joining Siemens Healthineers, I was assigned to work on a pioneering CFD/AI application, specifically focused on airways and lungs, to model airway diseases. This marked my introduction to the realm of artificial intelligence (AI). Conversations with colleagues and friends, whether over coffee, ping pong, or dinner, were brimming with enthusiasm about the potential of replacing cardiac CFD with machine learning models. Witnessing the efficiency of AI compared to traditional numerical techniques often left me exclaiming, ‘Wow, AI can do that?!’
From that point on, AI became a central component of my work. I integrated AI into my Ph.D. as well as into side projects and proactive initiatives during my full-time role at Siemens. As interest in AI surged within the engineering simulation community, I gradually took on more responsibilities. My time became divided between CFD for turbomachinery applications and AI. Collaborating on small side projects with developers interested in exploring AI paved the way for my involvement when customers began expressing interest. Eventually, recognizing the increasing significance of AI, an official AI position was established, which I happily accepted. And that brings us to my current role as a Senior AI/Machine Learning (ML) Technical Specialist in Product Management. In my current position, questions about AI have become increasingly prevalent across vendors and customers in the space. It’s remarkable to witness the evolution of interest in AI and its impact on our work.
Could you provide a brief overview of AI, machine learning, and Computational Fluid Dynamics (CFD)?
Humanity’s recent development of statistics and regression models in Computational Fluid Dynamics (CFD), my main area of expertise, has been remarkable. Whether it’s five, ten, or even thirty years ago, these techniques have played a crucial role in data-driven correlations, especially in CFD, Finite Element Analysis (FEA), and Computer-Aided Engineering (CAE). People use these methods to generate data, establish connections, and analyze results using straightforward math to understand design spaces.
Traditionally, practitioners employed these techniques to create and analyze data, using simple math to grasp aspects of design spaces. Machine learning operates on the same principles and aims for the same goals as traditional statistical methods but introduces a different approach that enhances non-linear capabilities, revealing intricate relationships within complex data sets. While the main goal remains the same, there’s a shift from the simplicity of linear regression, like what you might do in Excel. Machine learning allows exploration of complex relationships, going beyond linear classifications.
For example, starting with a basic linear regression like distinguishing between cats and dogs based on visuals or data (e.g. weight), you can move on to more complex scenarios. As relationships become more intricate, machine learning can predict complex outcomes, such as the likelihood of someone developing heart disease based on various lifestyle and hereditary factors. This analytical approach extends to computer vision models, identifying individuals in street photographs, and contributing to the training of autonomous vehicles.
These advancements bring in new paradigms, including large language models built on neural networks. AI becomes increasingly valuable in predicting complex outcomes, like full simulation results, and enhancing software capabilities. However, it’s essential to recognize that the foundation of these advancements can be traced back to regression analysis, and they follow the same principles, maintaining a significant overlap with statistical methods.
What is one common myth about AI or machine learning that you’d like to debunk?
AI cannot do everything, but it excels at some crucial tasks, performing at least 10 times better than the traditional approach. Contrary to popular belief, AI is not a new concept. Many methods are continually being developed by mainstream technology companies. Cross-pollination between different fields is improving, especially in applications like engineering simulation. These methods are becoming more robust, interpretable, and scalable. Recognizing this trend, people should consider investing in AI as it moves in this direction.
Do you have a favorite simulation that you created?
One of my recent favorite simulations involves our optimization software, where machine learning models are directly trained in the background during an optimization process or study. Traditionally, in CFD simulation software, one might aim to minimize a car’s drag for fuel efficiency and maximize its downward force, ensuring stability during tight turns. This process has been a standard practice for decades. Simultaneously, machine learning models are trained on the simulation data as the study progresses. The machine learning model gains autonomy by making decisions on whether certain points can be skipped, streamlining the process for accurately predicting the next point. This automated decision-making significantly reduces the time required. While traditional simulations can be expensive and time-consuming, taking hours or even days, the machine learning model provides predictions within seconds.
The integration of machine learning into our optimization processes is fascinating. What sets it apart is that it’s not just a theoretical concept; it’s actively being utilized in the industry through our software. This real-world application makes it one of my recent favorites, highlighting the practical application and impact of combining machine learning with optimization.
What is one lesson you have learned on the job, whether about technology or otherwise, that you believe everyone should know?
My understanding of emotional intelligence grew during an online product management class I took some time ago. In the fast-paced world of industry, where deadlines are tight and conflicts are common, it’s easy to overlook these interpersonal skills. While recognizing the importance of developing emotional intelligence is straightforward, putting it into action is quite challenging.
Effective collaboration in a group happens when each member reflects on how they interact with others, their habits, and their communication styles. This ensures alignment with the diverse array of personalities encountered. Being considerate and mindful of your colleagues and the context not only improves project outcomes but also adds a sense of enjoyment to your work. I genuinely value all my colleagues at Siemens and take immense pleasure in working with them. I see them not just as coworkers but as friends. Having genuine connections with people who are not only smart and talented but also interesting is a rewarding outcome.
How do you avoid feeling burnt out in your role?
It’s important to acknowledge that there may be times when you immerse yourself in work, engaging in substantial sprints fueled by passion. Occasionally, you might put in extra hours, even outside the regular work schedule, due to your enthusiasm and excitement about the project at hand. While these bursts of productivity are generally positive, it’s essential to monitor the long-term effects on work-life balance and potential burnout.
Similarly, you can intentionally maintain a moderate work schedule to ensure everything stays in check and remains balanced. Working modest hours is important because working overtime consistently is not sustainable. However, you can find a middle ground, adjusting your workload in moderation based on your excitement and energy levels.
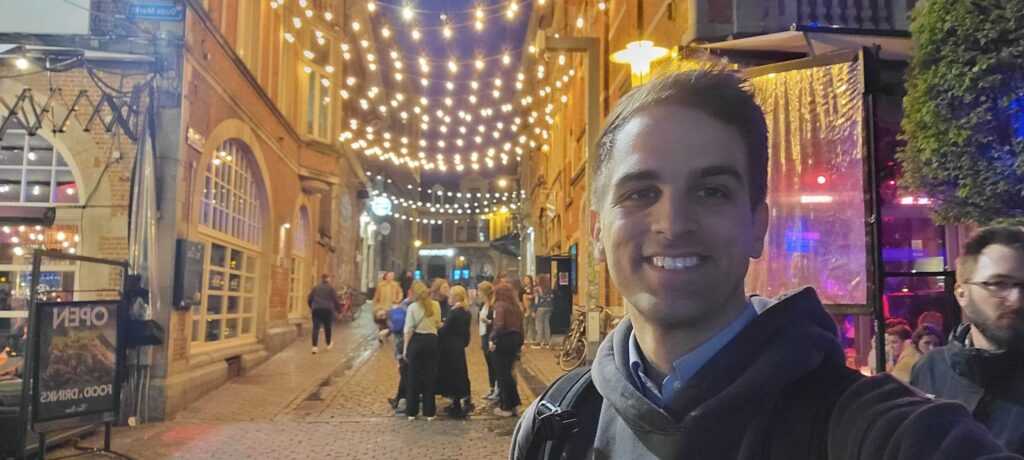
Do you have any career advice for interns or students starting out in the workplace?
I strongly believe in the value of having a mentor. Throughout my career, I’ve been fortunate to have had three incredibly impactful mentors who have played a significant role in my intellectual growth. This belief aligns with the perspective of David Sinclair, a renowned geneticist and professor at Harvard Medical School, who emphasized the importance of continuous practice and growth in intelligence over time. Personally, I consider having a mentor an important element in achieving this, especially since I perceived myself as average during my school years. However, by staying committed and intentional in my pursuits, I’ve seen improvement.
Another piece of advice I advocate is setting ambitious goals, particularly when establishing personal objectives. Including a subset of lofty goals can lead to surprising achievements and serves as a powerful motivator to maintain momentum. Lastly, I emphasize the significance of networking and building relationships. Life doesn’t guarantee a constant supply of special individuals, so it’s essential to be mindful of fostering connections. Whether as a colleague, friend, or in any other role, actively contribute to maintaining meaningful relationships throughout your life, because relationships are special.
Quick takes
If you could have a superpower, what would it be?
My superpower would be teleportation, so I don’t have to waste time driving places. I could just be there, do what I need to do, and then come back.
Do you build what you create, or do you create what you build?
You create what you build. I would think what you created was conceptualized, planned, and realized that an intention or project would work and be feasible. Then the building is executed.
What’s something that people would be surprised to know about you?
I have a couple of them. First, I love my job. I am constantly reading and learning things related to AI. Second, I think the fulfillment and intention of being a good father are so much more than that. I’m sure other dads can relate, but that’s a big one. The last one is not as surprising, but I love making espresso at home. My colleagues quickly learn this about me whenever we start learning about each other and working together. I am a fanatic for espresso. I roast my own beans at home, grind them, and dial them in with my machine. My espressos are meticulously prepared and made. That’s something that I really like that people eventually learn about me when we hang out enough.
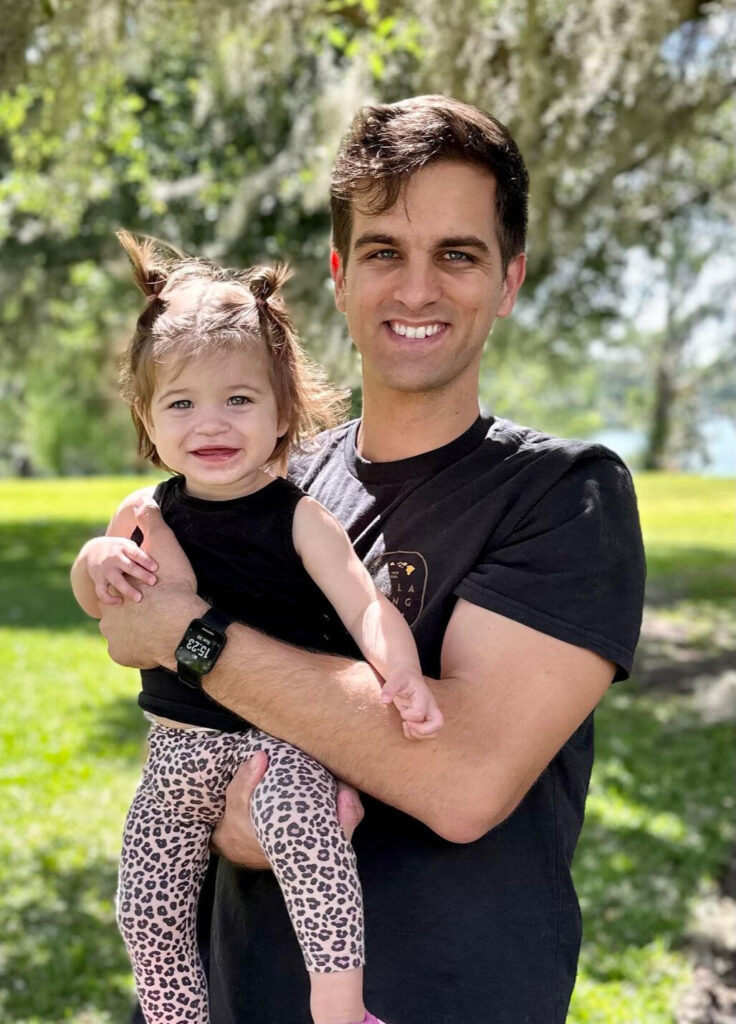
Do you have any book, movie, or song recommendations?
I recommend the book “Man’s Search for Meaning” by Victor Frankel. It’s about how to go through hard things and overcome them while keeping a positive mindset of gratitude. The book touches on Frankel’s personal experience of hardship, along with how he adapted his mindset to overcome it. The second half of the book touches on clinical psychology and ties it to his teachings and learnings in the field.
If you could eat one food for the rest of your life, what would it be?
I was vegan for five years, but my answer is definitely steak and potatoes.
Check out Justin’s new book, “Approaching machine learning problems in computational fluid dynamics and computer aided engineering applications: A Monograph for Beginners“ on Amazon!
About the book
This is not a conventional textbook or reference text (closer to a monograph). This is an abstraction of experiences into a practical guide to get CFD/CAE practitioners more comfortable in machine learning projects. After hundreds of requests for support, I felt the conviction to set aside my nights for 6 months and produce this book as a more scalable means to help.
This book has a lot of code (not shareable on Github). There is an abundance of resources that cover theoretical knowledge of machine learning in ‘the mainstream’, but relatively little by comparison for CAE applications (especially few that are hands-on). My hope is that the reader already has some (very minimal) theoretical knowledge when they pick this book up. There will be some explanation on the algorithms with examples (in Python), and some degree of surveying/summarizing popular ones, but the primary focus is how and what you should do to solve machine learning problems. This is what I refer to as the pipeline of steps from start to finish in a machine learning project, which seems to have a steep learning curve (my motivation for writing this book). This book will also share my recommended learning pathway for CFD/CAE engineers to develop their AI/ML skills and portfolios and is great for beginners.
I am a fan of the ‘code along’ approach and take that to heart in this book. I recommend reading the book while logged into a computer where you can code.