Exploring Hurricane and Semiconductor Dynamics: Modeling, Simulation and Predictability.
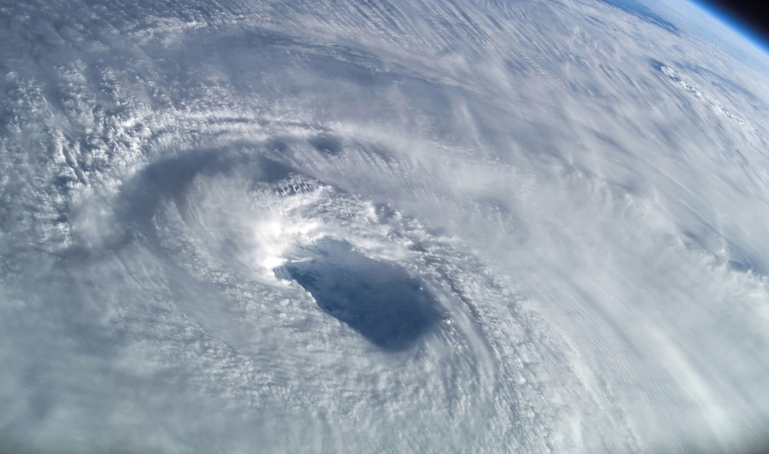
It’s that time of year again – hurricane data season is upon us.
Twenty-first century meteorologists still rely on the hurricane hunter aircraft that flies directly into and through the storm to collect vital measurements of the storm characteristics. The information is then transmitted back to the meteorologist who updates their weather models and reruns their simulations to get the latest forecasts for the hurricane. The measurements collected range from atmospheric pressure and temperature, from wind speed and humidity, are just some of the data used to provide a comprehensive snapshot of the hurricane’s current state and future path.
Additionally, hurricane forecasting relies on a dizzying array of data sources beyond hurricane hunter aircraft, such as satellite observations, oceanic buoys and sensors for water temperature and wave heights. Other information comes from weather fronts and air masses, jet streams, weather stations and weather models of the current weather conditions in the projected path of the hurricane.
Collecting, analyzing and interpreting data sources is crucial for accurate hurricane modeling and simulation, and there are significant challenges associated with integrating, and processing this information. The key challenges include:
- Data quality and consistency: Ensuring the quality and consistency of data from different sources can be challenging. Variations in measurement techniques, calibration and reporting standards may introduce errors or inconsistencies that can impact the accuracy of the simulation models.
- Data resolution: The resolution of data—both spatial and temporal—is a critical factor. Higher-resolution data allows for more detailed modeling but can be computationally demanding. Balancing the need for precision with computational efficiency is an ongoing challenge.
- Data assimilation: Integrating data from various sources into numerical models requires sophisticated assimilation techniques. Ensuring that observations are effectively incorporated into the models, without introducing biases, is a complex task.
- Incomplete data coverage: Some regions, especially over oceans, may have limited data coverage. Remote and uninhabited areas can lack weather stations, buoys, or other observational tools, making it challenging to gather comprehensive information, especially in the early stages of storm formation.
- Rapid changes in hurricane dynamics: Hurricanes can undergo rapid changes in intensity, direction and structure. Real-time data collection may struggle to keep up with these changes, particularly if the sampling frequency is not high enough.
- Instrumentation limitations: Instruments on satellites, buoys
,and other observation platforms may have limitations in their capabilities. For example, certain sensors may not function optimally in extreme weather conditions, limiting their effectiveness during the most critical phases of the storm. - Complexity of atmospheric processes: The atmosphere is a complex system with numerous interacting processes. Simulating these processes accurately requires comprehensive understanding and modeling capabilities, and uncertainties can arise due to the inherent complexity of atmospheric dynamics.
- Model initialization: Starting a simulation with accurate initial conditions is crucial. However, uncertainties in the initial conditions can propagate throughout the simulation, affecting the accuracy of projections. This is especially challenging in the case of rapidly intensifying hurricanes.
- Predictability limitations: Despite advancements, there are limits to the predictability of certain aspects of hurricanes, such as the exact timing and location of rapid intensification events. This inherent uncertainty poses challenges in providing precise projections.
- Computational resources: Running high-resolution models and assimilating vast amounts of data require substantial computational resources. Access to powerful computing infrastructure is essential for conducting simulations at the necessary scale and speed.
Addressing these challenges requires a combination of technological advancements, improved observation networks, enhanced modeling techniques, and ongoing research to better understand the complex dynamics of hurricanes.
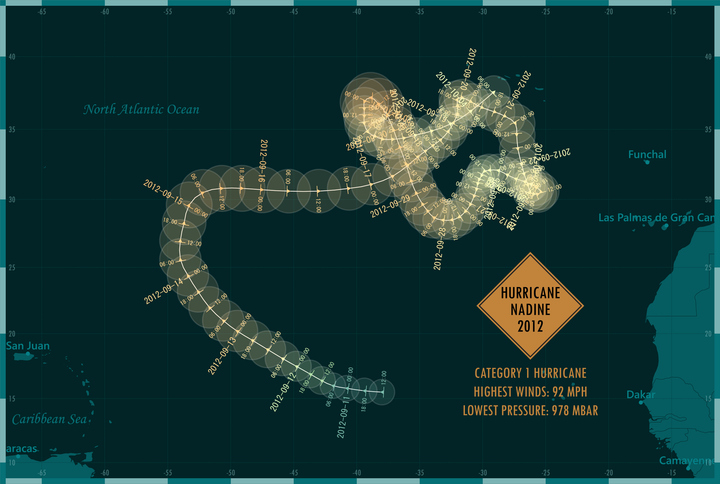
“Is this how you feel trying to make sense of your semiconductor manufacturing data?”
Continuous streams of data are essential to further improve the accuracy of hurricane projections and enhance preparedness for these natural disasters.
However, getting a continuous stream of true real-time information is the biggest challenge that has yet to be resolved. Hurricane hunter aircraft cannot continuously stay within a hurricane throughout its lifecycle. Logistical challenges associated with such a mission, including fuel constraints, potential damage to equipment from extreme weather conditions, and operational costs prohibit from streaming real-time data, that would lead to significantly improved hurricane projections and predictions.
The key benefit of continuous, high-frequency data feedback is the ability to capture the dynamic changes within the storm as they occur.
Moreover, with the rise of AI, the art and science of hurricane simulation is progressing rapidly, and even semiconductor giants like NVIDIA are exploring new ways to advance hurricane simulation and visualization – nvidia-enhances-climate-research-with-new-simulation-capabilities/
Now, instead of collecting real-time data being consumed by the models used to simulate hurricanes, how would semiconductor manufacturers benefit having real-time wafer fabrication measurements being immediately streamed back into the Digital Twin data model that engineers use to simulate the current semiconductor fabrication process actively running on a fabrication line in that facility?
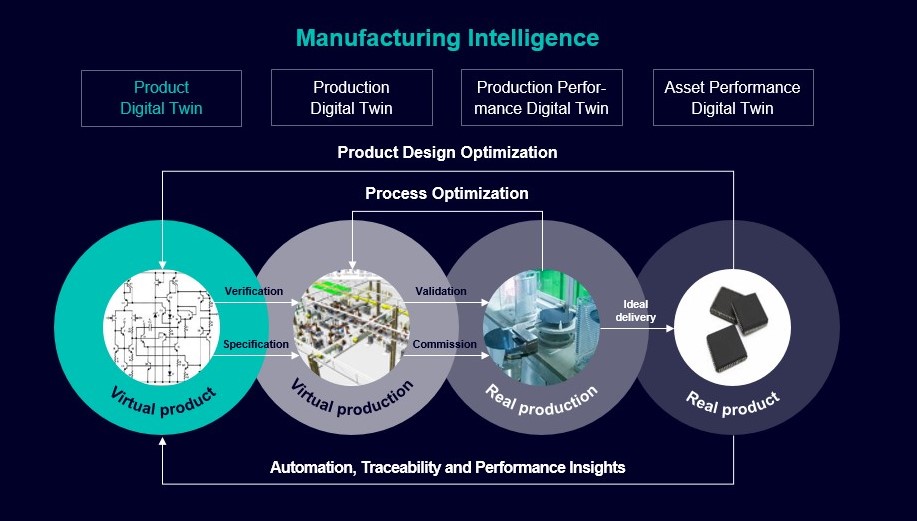
If semiconductor manufacturers could integrate real-time wafer fabrication measurements into the models used to simulate the semiconductor fabrication process, it could have several significant benefits for the industry:
- Process optimization: Real-time data would provide an instantaneous and detailed view of the fabrication process, allowing engineers to identify and address issues promptly. This could lead to more efficient and optimized processes, reducing waste and improving overall yield.
- Quality control: Continuous monitoring of wafer fabrication measurements in real-time would enhance quality control capabilities. Any deviations or anomalies in the manufacturing process could be quickly detected, enabling immediate corrective actions, and ensuring higher product quality.
- Fault detection and prevention: The ability to stream real-time data into simulation models would enable the detection of potential faults or anomalies before they escalate. This proactive approach can help prevent costly production interruptions and minimize the risk of defective semiconductor components.
- Rapid process adjustments: Real-time data integration allows for rapid adjustments to the fabrication process based on live information. This agility is crucial in an industry where small variations in conditions can have significant impacts on the final product.
- Energy efficiency: Continuous monitoring and real-time feedback could contribute to more energy-efficient processes. By optimizing parameters based on immediate measurements, semiconductor manufacturers can reduce energy consumption and environmental impact.
- Predictive maintenance: Real-time data analytics can be leveraged for predictive maintenance of manufacturing equipment. By monitoring various parameters, manufacturers can anticipate equipment failures and schedule maintenance activities, minimizing downtime and extending the lifespan of critical machinery.
- Cost reduction: Enhanced process efficiency, quality control, and fault prevention can collectively contribute to cost reduction. Minimizing errors and optimizing production parameters in real-time can lead to resource savings and improved overall economic performance.
While the concept of real-time integration into simulation models such as Digital Twins offers numerous advantages, it’s essential to address challenges such as data security, system integration complexities, and the need for robust and reliable sensors.
Additionally, secure collaboration between semiconductor manufacturers, simulation model engineers, and data scientists is crucial to developing effective frameworks that seamlessly merge real-time data and simulation models in a manufacturing environment.
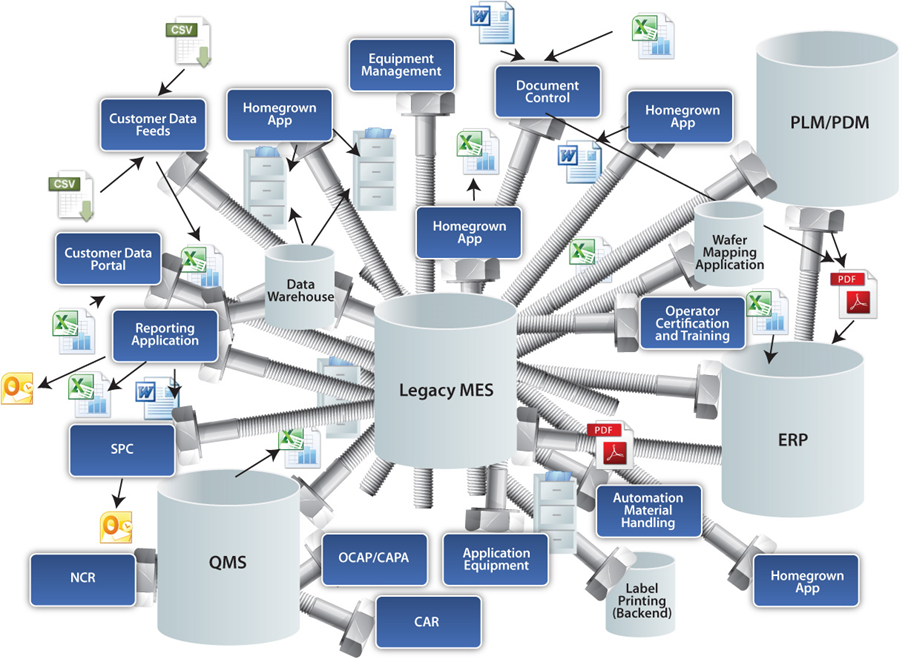
“Is this how you plan to manage your semiconductor manufacturing data?”
Even when the semiconductor fab is operating within its process tolerance ranges, updating the virtual models based on changes in real-time measurements, even within tolerance levels, can have significant implications for maintaining or improving the quality and yield of products in semiconductor fabrication.
For example, consider a scenario where, over the past three weeks, real-time measurements in a semiconductor fabrication process have consistently hovered just above the lower threshold. Suddenly, there is a shift, and the measurements now reach the upper threshold. In such a situation, would collecting this information and updating the models for that specific stage of the semiconductor fabrication process potentially enhance or maintain the quality and yield of the products currently undergoing production? This question holds even if the measurements, despite the shift, still fall within the acceptable tolerance levels.
Here’s why:
- Optimizing parameters: Shifting from measurements at the lower end to the upper end of the tolerance range may indicate an opportunity to optimize process parameters. By updating the models to reflect these variations, engineers can fine-tune the manufacturing process, potentially achieving better performance, higher yield, and improved product quality.
- Preventing drifts and deviations: Even if measurements remain within tolerance levels, detecting a shift from the lower to the upper range is valuable. It could be indicative of subtle changes in the production environment or equipment performance. Updating the models allows for proactive adjustments to prevent further drifts and deviations, maintaining stability in the fabrication process.
- Quality assurance: Real-time adjustments based on changes in measurements help ensure consistent product quality. By promptly responding to variations within tolerance limits, manufacturers can uphold stringent quality standards and reduce the likelihood of defects or inconsistencies in the final semiconductor products.
- Early warning for issues: A change in measurements, even if within tolerance, can serve as an early warning system. By integrating this information into the simulation models, manufacturers gain insights into the evolving dynamics of the fabrication process. This early awareness enables proactive problem-solving, preventing potential issues before they escalate.
- Continuous improvement: Semiconductor fabrication is a complex and precise process. Real-time feedback allows for continuous improvement by incorporating the latest data into the simulation models. This iterative approach supports ongoing optimization and ensures that the manufacturing process remains at the cutting edge of efficiency and quality.
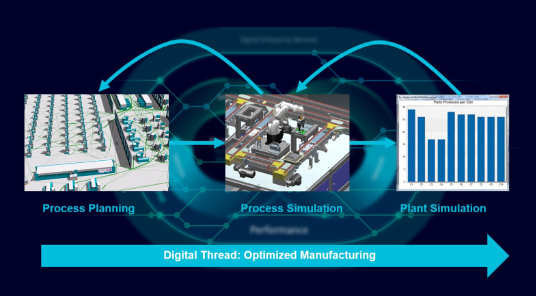
In summary, even subtle changes in real-time measurements within tolerance levels provide invaluable insights that, when incorporated into simulation models, empower semiconductor manufacturers to fine-tune and optimize their processes. This proactive approach not only helps maintain product quality but also creates opportunities to build upon proven runs for continuous improvement, and progressive innovation in semiconductor fabrication.
For more information about semiconductor simulation and the emerging role of the Digital Twin in semiconductor manufacturing, see our informative eBook: e-book-digital-twins-the-cornerstone-of-smart-semiconductor-manufacturing
Or visit David Corey’s insightful Blog for more about the evolution of Executable Digital Twins (xDT) in semiconductor fabrication: www.linkedin.com/pulse/executable-digital-twins-replacing-advanced-process-david-corey-ksvbe/