Saving the day with simulation knowledge management for Teamcenter
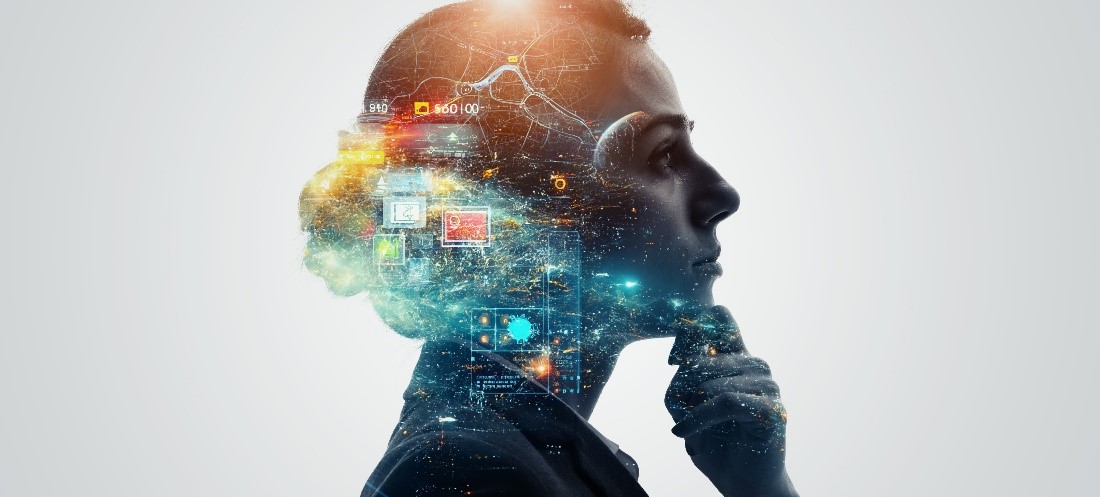
Joining a brand new environment
Sam has just started her new job as a simulation engineer at a large engineering company. There are many perks: bigger team, friendlier people, and the coffee – equally important – is excellent. She loves it already!
One Friday afternoon, her manager comes into her office and has an urgent request. The design of a product, the team has been working on, has a slight, last-minute change (new supplier, slightly different material properties), and needs a new analysis. Her manager expects results before the weekend: „Running the analysis does not take long. Simply reuse one of the existing models, change the parameters, and rerun the simulation”.
Reuse – Change – Rerun
Right after her manager leaves, Sam feels a rush of blood to her head, a hint of panic she hasn’t felt in a while. Reuse? Rerun? “What is he talking about?” She has no idea how to find the right model. Yes, the company manages a simulation model database and every team member is supposed to store the models there together with detailed documentation. She hastily connects to the database, but what she sees does not help at all: the documentation is scarce at best, and the file names are hieroglyphs to her. Her new colleagues are fun but clearly do not invest much effort in properly documenting and annotating their work.
She quickly stands up, walks around the office, but no one is there. Everyone has left for the weekend. She is alone (except her manager), and no one there to ask. She feels small and incompetent: “I will have to do the analysis from scratch, and it will become obvious how slow I am. There is no way that I can finish that work before the weekend.” Full of self-doubt she plods back to her desk.
SPDM
Still, she is determined to do as much as she can. Following the best-practices of her new company, she starts to prepare a new simulation request within the SPDM system, which includes the information provided by her manager about the materials, CAD design, and boundary conditions. Now it is time to defeature the geometry, generate a good mesh, specify the boundary conditions, and loads before finally running the new simulation. And then there is also the question of defining a new material. She really can’t remember how that was done exactly.
The simulation request is finally set up when Sam notices a tab called Recommended Simulations. “Interesting”, she mumbles to herself. She is curious.
Recommended Simulations
When she clicks there, she is presented with a list of simulation files related to the job she has to do, many of them even using the same CAD file. “What? How?” she stutters in disbelieve. “Can that be real?”, and she starts to understand that a large part of the work does not need to be redone. Still puzzled, she scrolls down the list and even finds a different model in which the user has changed the material properties. From this file she can probably learn how to define a new material for her task.
Sam feels her energy coming back. Maybe she will manage to deliver the results before the weekend. She still wonders how the system was able to recommend her the right simulations. Was this mind reading?
Knowledge graph
Definitely not! This is how we envision recommendation engines boosting productivity of engineers in the future. The technology powering this recommendation engine? A knowledge graph. More concretely, all the simulations in the database are processed and simulation metadata is extracted automatically. An ontology has been defined to connect the metadata in a meaningful way. Regularly new simulations are processed, metadata extracted and fed to the knowledge graph. The resulting graph contains all the simulation knowledge from the database, which can be queried to provide the desired information. For the recommendation engine, a similarity criterion is defined, and the user is shown a list of “similar” simulations. If the model is nearly the same, it can be used as basis for further analysis. Otherwise, the user can leverage the provided recommendations from the database to learn best practices from colleagues on how to choose simulation parameters, how to apply boundary conditions, define new materials or run a specific type of analysis according to the company guidelines. Investing significant amount of research and predevelopment into this as well as other exciting new technologies, we make sure to keep increasing productivity significantly.
And now back to Sam
She has a look into the simulation to learn how to define a new material and reproduces this change in the model with the same CAD. She runs the simulations, and the results look good.
“Wow. Done in a jiffy. No wonder why everyone could leave so early for the weekend.” Simulation knowledge management saves a lot of time, ensures high quality and – what Sam has experienced today – helps to learn best practices.
Before Sam heads out for the weekend, she thinks this deserves a little celebration. So, she stops by the employee kitchen to grab one last cup of coffee for the work week. When her manager passes by to check-in and wish her a nice weekend, she can’t hide her smile… “Yeah, just like you said, reuse, change, rerun… Nice one to you too.”
“Excellent…” she thinks. “…not just the coffee!”